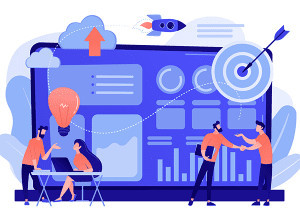
Businesses succeed or fail based on their ability to make sound decisions. Every aspect of an organization’s operation, from the simplest to the most complex, is the result of a series of decisions, each building on the next, from the chairs in the employee lunch room to the million-dollar marketing campaign that will make or break the operation.
While business instincts and gut feelings may factor into a company’s decision-making, it’s much more likely that the choice between available options will be based on information relevant to pertinent factors, such as goals, pricing, technology, and the pros and cons of each alternative. Yet having too much of the wrong information can be as bad or worse than having too little of the right information.
Data-driven organizations base their decisions on the analysis of information that they have confirmed is accurate, relevant, complete, and timely. Simply collecting massive amounts of data can hinder rather than assist in making sound business choices, however. To be truly data-driven, a company must reimagine its entire operation so that its data is democratized, or made available to all employees rather than merely to data analysts.
A History of Data-Driven Business Decisions
The use of data to support business decision-making grew popular in the mid-20th century, when researchers described the process of making decisions as dynamic, complex, and potentially ambiguous. They determined that the uncertainty and risk endemic to decision-making could be measured and mitigated. Computer-aided decision-making arrived in the 1970s, but early techniques focused on decision models, such as preference trees (commonly referred to as “decision trees”) and prospect theory.
Data first took a leading role in business decision-making in the 1980s with the arrival of commercial decision support systems. The importance of information systems increased in the early 21st century as data warehousing and data mining expanded the potential of data analytics. The effectiveness of these massive data stores was limited by the inability of companies to manage the information due to a lack of clear organization-wide policies and guidelines.
The goal of modern data-driven decision-making is to improve the quality of business decisions by combining the capabilities of automated decision models with the expertise, creativity, and critical thinking of human managers. Achieving this goal requires leveraging the work of data scientists in putting data-powered decision-support tools in the hands of managers and employees in all areas of the business.
What Makes an Organization Data Driven?
A data-driven organization understands the value of its data and the best ways to capitalize on that value. Its data assets are aligned with its goals and the processes in place to achieve those goals. Protecting the company’s data assets requires incorporating governance practices to ensure managers and employees abide by privacy, security, and integrity guidelines.
In addition to proper data governance, the challenges to implementing a data-driven infrastructure for business processes are data quality and integrity, data integration, talent acquisition, and change management.
- Data quality and integrity for data-driven operations requires continuous monitoring of data based on rules established by the business. Automated checks are used to spot and address potential errors or other threats to the data’s value and usefulness.
- Data integration involves three processes for combining data from diverse sources to create a single, unified repository providing a consistent view of the data: extraction (consolidation) into a single system, transformation of various formats and structures, and loading into a central repository. An ETL pipeline is a type of data pipeline that terminates once the data has been added to the repository.
- Talent acquisition becomes as data driven as the organization’s other operations by applying the same analytics tools to information collected on potential and actual job candidates. Companies are able to identify top applicants faster and get them onboarded more quickly.
- Change management benefits from data-driven approaches by allowing companies to identify the most effective incentives for employees, enhance their autonomy, and help them acquire new skills. The rewards to individuals help establish a sense of shared cultural values that connect departments and operations companywide.
What Are the Benefits of Being a Data-Driven Company?
Businesses understand that becoming a data-driven company requires more than throwing hardware and software at problems and expecting immediate results. To ensure the success of their increasingly critical data initiatives, organizations look to the characteristics that led to effective adoption of data-driven programs at other companies.
Management services firm KPMG identifies four key characteristics of successful data-driven initiatives: leadership involvement, investments in digital literacy, seamless access to data assets, and promotion and monitoring.
- Leadership involvement is critical to the success of data-driven programs from day one by impressing on managers and employees the importance of data to their work. Company leaders are encouraged to cite real-world examples of how data-driven strategies have helped other organizations.
- Digital literacy and tools expand the scope of data literacy to encompass cloud technologies, AI-based analytics, and automated work processes. Initiatives to teach new skills to managers and line workers are continuously evolving to meet shifting governance and data quality requirements.
- Seamless access to data assets ensures that companies realize the full value of their investment in digital literacy tools and training. Data marketplaces support intelligent searches of data stores using analytics dashboards that are easy for non-data experts to customize to their needs. They also centralize role-based access control and governance, and confirm the fitness of the data for its intended purpose.
- Promotion and monitoring of the data-driven initiative are as important after implementation as during the process. The availability of data and analytics tools and the benefits they provide evolve as technology, market and industry conditions, and the organization’s own internal and external processes change over time.
Data-driven corporate cultures support faster and more effective decision-making and also enhance employee engagement and the business’s overall value. They integrate data ethics as a core component of digital literacy by highlighting the negative impact of data bias and providing tools for identifying and remediating bias and other anomalies that reduce data quality.
How Does a Business Become Data Driven?
Much of the impetus for organizations to be data driven results from the promise of generative AI, which McKinsey estimates will add between $2.6 trillion and $4.4 trillion of value to the global economy each year across 63 use cases. A full 75% of this value will be realized in four areas: customer operations, marketing and sales, software engineering, and research and development.
Making the transition to a data-driven company entails shifts in an organization’s relationship with its data:
- Adopt a data first approach. Define clear goals for breaking down data silos and democratizing access to data assets. Analyze your existing data infrastructure to identify upgrades required to achieve your data goals. Ensure that everyone in the organization, from the C-suite to front-line positions, are involved in the planning, implementation, and management of data-first processes.
- Take advantage of data and analytics technologies. These include real-time data insights, as well as environmental, social, and governance (ESG) reporting in light of new mandatory disclosure requirements. Increased reliance on AI heightens the need for integrating ethical and societal safeguards in newly automated data processes.
- Focus on dynamic and reusable data products. The shift from using custom data sets in development projects to multi-purpose data products that are easy to adapt to specific circumstances reduces the need for data engineering and enhances return on investment by getting products to market faster.
- Treat data like a product. While data-as-a-service (DaaS) emphasizes the sale of external data, data as a product (DaaP) considers all of a company’s data and the mechanisms in place for moving and storing the data as a product that internal operations rely on. The data team becomes a “vendor” serving “customers” throughout the organization.
- Make chief data officers revenue generators. Rather than spending their time reacting to data inefficiencies in their companies, chief data officers (CDOs) have to prioritize delivering business value. The business units that create the data take responsibility for managing and maintaining it based on strict company-wide governance standards. This allows CDOs to devise strategies for optimizing the value of the organization’s data assets.
- Implement a data-sharing infrastructure. An integrated data ecosystem combines all the data tools, frameworks, interfaces, and policies, as well as all operations involving the collection, storage, processing, and use of the data. This eliminates data silos and promotes safe and timely access that maximizes the value of data assets.
- Automate and prioritize data management. Companies are turning away from single-purpose data management tools in favor of unified platforms that simplify the data stack and make it easier to prioritize the organization’s most valuable data assets. The platforms automate data quality monitoring to resolve quality issues quickly while being easy to scale to allow their use for diverse purposes across departments.
Becoming a data-driven organization prepares a company for automated decision-making using no-code interfaces and models capable of supporting complex decision logic. Data travels fluidly between CRM, ERP, and line-of-business applications with little or no need for preprocessing. These systems empower rather than replace human decision-makers, allowing managers to apply their creativity, experience, and intuition to the highest quality data available.