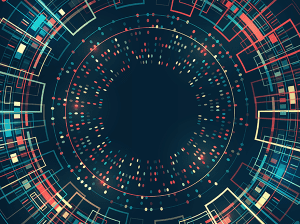
Data silos represent a major business challenge, as noted by 60.9% of organizations in a recent Trends in Data Management survey. Without shared information, companies risk duplication, poor data quality, and missed opportunities for innovation. Consequently, many companies turn to modern and integrated data architectures. When doing so, organizations often consider two main approaches: data mesh and data fabric. Data mesh follows a decentralized sociotechnical approach to sharing, accessing, and managing analytical data. Data fabric, on the other hand, unifies disparate data across systems through data pipelines and good metadata.
Both data mesh and data fabric aim to break down data silos. However, they take different approaches to achieve this goal. Moreover, some businesses find that implementing just one of these architectures in isolation can inadvertently lead to the growth of new data silos.
A good solution lies in combining data mesh and data fabric into a hybrid architecture. To do this effectively, organizations first need to understand the basics of each approach and how they can work together.
What Is Data Mesh?
Data mesh integrates information through a self-service, collaborative data platform. Business units across the enterprise control data services and their products composed of datasets. This feature breaks down silos.
To promote sharing and standards, data mesh architectures rely on federated data governance (DG). This type of governance program increases engagement across teams and creates data products for the company to sell.
By working together to establish shared standards and best practices, teams across the company see improved data quality and optimized resources, which is necessary for effective data integration. In doing so, businesspeople have more self-sufficiency with their data and break down silos caused by bottlenecks.
While data mesh can break down silos, its complexity can lead to challenges. It requires a lot of domain knowledge and technical expertise, resources, and consistency to function. Each business area requires IT and Data Governance support for two key aspects: maintaining the architecture and ensuring well-defined data roles and processes.
A Data Mesh Example
Despite its complexity, data mesh does effectively make data more accessible. For example, a leading manufacturer had over 230 data silos across 30 countries. By adopting this decentralized approach, this manufacturer united fragmented data ecosystems through the creation of a data marketplace. The company found this implementation flexible and scalable. It had domain-specific data products that could be accessed across locations, which led to new capabilities.
What Is Data Fabric?
While the manufacturer’s success with data mesh is impressive, not all companies have the resources or organizational structure to implement such a decentralized approach. Instead, they turn to data fabric, which uses technology to make data accessible in one place.
In its simplest form, a data fabric takes in metadata from participating systems and users, analyzes it, produces alerts, and makes recommendations for better data integration and business support. Moreover, this architecture builds on a combination of AI, automated algorithms, and polyglot persistence, a feature allowing flexibility in coding language. Consequently, data fabric pieces together different systems regardless of their integration styles or storage functionality. See the figure below.

Data fabric breaks down silos through a data virtualization-like experience. Consumers find and obtain data all in one place, reinforced by centralized data governance. This architecture makes organizational data a reusable resource to augment, refine, and resolve per use case.
At the same time, a data fabric architecture may face resistance to sharing data through the physical layer that connects different systems and sources. To share, teams must give up control to a centralized department handling the data fabric. Businesses may be unwilling or unable to do so, resulting in siloed information.
A Data Fabric Example
Despite potential bottlenecks, data fabric does improve decision-making. Domino’s implemented this architecture to unify multiple architectures in one place. Domino’s data fabric integrated and unified a large, distributed data ecosystem. Consequently, the company tracked food and delivery data from point-of-sale systems, supply chain centers and across all marketing campaigns.
Combining Data Mesh and Data Fabric
Clearly, data fabric shines in technologically bringing together disconnected data, but data mesh capitalizes on self-service. Combining both architectures skillfully improves data discoverability and access consistently through data fabric, while increasing agility and innovation through data mesh.
Determining the best hybridized version requires understanding business objectives and how a data strategy, with the overarching architectures, needs to support these goals. Key considerations include:
- Assessment of data capabilities: Organizations need to measure how well they use data given the current strategy, architecture, technology, and organization. Then they need to think about their next steps. A business facing multiple data silos, resistance to change, and utter chaos may want to minimize upgrading the data architecture and work on standardization.
- Culture: As Peter Drucker said, “Culture eats strategy for breakfast.” If a technology company has a decentralized business structure, where each team owns its data, then first building out a data mesh architecture may make sense before combining data fabric. Improving automation capabilities through a data fabric, as a reaction to a failed data mesh implementation, will not lead to data sharing.
- Use Cases: Corporations need to consider use cases that are good candidates for trying a melded mesh/fabric approach. Also, the business needs to continue to generate revenue and customer satisfaction. Consequently, organizations need to consider the resources available to blend data mesh and data fabric and those required to keep operations running.
- Data Governance: Making changes to the data architecture by adding more mesh or fabric will impact governance. For example, if a company has a formalized governance program with training programs, then adding more data mesh will add complexity, frustration, and silos. Research how the data governance policy, the security and data quality standards, and its implementation will impact the existing program.
- Technical Resources: The existing infrastructure and skill sets available will inform how to hybridize data fabric and mesh. For example, a data mesh will require event handling for systems to combine data products and synchronize activities around them. Building this kind of ecosystem from scratch, without enough skilled IT, will result in less success.
- Continuous Improvement: Figuring out the best combination of data mesh and fabric requires methodical and continuous improvement. Companies need to try a small change, collect objective information based on metrics, and ensure repeatability of results over the long term. Silos may emerge for a project for a small period, but the overall number should decrease.
Benefits and Challenges of a Combined Architecture
Even a thoughtful deployment of hybrid data mesh/fabric, which accounts for different key factors, has its benefits and drawbacks. On the plus side, companies have easier access, regardless of location, and more controlled security protocols.
In the meantime, these companies reap the benefits of self-service and flexibility from a data mesh decentralized approach. Combined skillfully, data sharing happens because data is easier to find and retrieve, without the bureaucratic headaches of having to submit a form to another group to get the data.
However, setting up a combined data mesh and fabric architecture does come with challenges. This framework is more complex than either alone or requires cultural changes. Business teams may win control of some datasets and may find additional processes and work for their data to be shared.
Then companies face unexpected silos. Teams become isolated in data mesh or circumvent using automation from data fabric, for departmental tools. These silos can further proliferate if an organization grows, or contracts and the hybrid architectural approach is not rebalanced.
Conclusion
Many businesses struggle with centralized or decentralized approaches to managing data. To solve this issue, they may turn to either data mesh or data fabric as a solution.
However, while companies find benefits to either approach, they can also experience the frustration of more silos. Business culture rarely conforms to either a purely formal or informal paradigm, including in their data activities.
So, organizations would do well to consider a hybrid approach that applies the best of mesh and fabric architectural philosophies in managing data. Having the best of both worlds addresses the need to get disparate data in one place while having the flexibility to experiment with datasets and address business demands in real time.
But a hybrid implementation needs the appropriate resources to break down the silos. With consideration of the time, money, and commitment to evolve to a mesh/fabric combination, increased data sharing becomes more feasible.