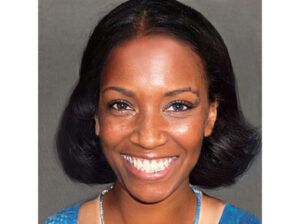
The increase in the prevalence and complexity of data breaches points to one conclusion – traditional cybersecurity measures are no longer enough to keep our valuable data safe. According to a recent report, data breaches exposed a staggering 35 billion records in the first four months of 2024.
To deal with this escalating crisis, a new solution is emerging: cybersecurity data lakes, which have the ability to consolidate and analyze vast amounts of data from various sources to provide a comprehensive and real-time view of an organization’s security preparedness, enabling early detection and prevention of potential threats.
Understanding Cybersecurity Data Lakes
A cybersecurity data lake is a centralized repository that ingests and stores an organization’s security-related data from various sources, including network logs, application logs, endpoint data, threat intelligence feeds, and more.
Its purpose is to enable comprehensive analysis, threat detection, and rapid response to cyber threats by providing a unified view of the organization’s cybersecurity posture. At the heart of a cybersecurity data lake is a scalable and flexible architecture designed to handle large volumes of diverse data. This architecture typically includes the following key components:
- Data ingestion layer: This layer collects data from various sources, such as network logs, security devices, and threat intelligence feeds.
- Data storage layer: This layer uses scalable and flexible storage solutions, such as Hadoop or cloud-based storage, to accommodate the vast volume of data.
- Data processing layer: Advanced processing capabilities, including machine learning and artificial intelligence, are applied to the data to identify patterns, detect anomalies, and generate actionable insights.
- Data access layer: This layer provides secure access to stored data for analysis and reporting. It includes tools for querying and visualizing data, enabling security teams to quickly respond to emerging threats.
Benefits of Cybersecurity Data Lakes
Cybersecurity data lakes have the ability to consolidate diverse data sources into a centralized repository. This integration process involves ingesting data from a wide range of sources, including network devices, security appliances, endpoints, applications, and threat intelligence feeds.
Cybersecurity data lakes can ingest both structured and unstructured data. Structured data, such as log files, system events, and database records, typically adheres to a predefined schema and format.
On the other hand, unstructured data, like email messages, social media feeds, and multimedia content, lacks a consistent structure and requires more advanced processing techniques.
While the prospect of consolidating all this data may seem daunting, it is essential for organizations that want to gain a comprehensive view of their cybersecurity readiness. However, the process of data ingestion and management comes with its own set of challenges.
First, the sheer volume of data generated by modern organizations can quickly overwhelm traditional storage and processing systems, necessitating scalable and efficient data ingestion pipelines.
Organizations can manage this by leveraging automated data ingestion pipelines to streamline the process and reduce manual effort. They can also employ distributed and cloud-based architectures that can handle large volumes of data and scale as needed.
Additionally, with data coming from diverse sources and in various formats, ensuring consistent data transformation and normalization can be a significant challenge. To address this, organizations need to implement a structured data annotation process to standardize and label the ingested data before feeding it into the data lake.
Threat Detection and Analysis with Data Lakes
Another advantage of data lakes is their ability to support real-time data ingestion and analysis. As security events occur, data streams are continuously fed into the data lake, enabling security analysts and automated systems to monitor and analyze them as they unfold.
This real-time visibility allows for rapid identification and mitigation of potential threats, minimizing the risk of data exfiltration or system compromise.
However, the true power of cybersecurity data lakes lies in their ability to harness advanced analytics and machine learning techniques. By combining structured and unstructured data from various sources, data lakes provide a fertile ground for sophisticated algorithms to uncover hidden patterns, correlations, and anomalies that may indicate potential threats.
AI models can be trained on historical security data to learn standard behavior patterns and establish baselines.
When new data is ingested, these models can detect deviations from the established norms and flag potential threats or anomalies for further investigation. This proactive approach to threat detection enables early intervention and prevention of potential breaches.
Training AI models often requires significant computational resources. To meet these demanding computational requirements, organizations might consider leveraging GPU server hosting instead of on-site servers, which can accelerate the training process and enable more sophisticated models for threat detection.
Advanced analytics techniques such as graph analysis, natural language processing, and deep learning can also be employed to gain deeper insights from the vast troves of data stored in cybersecurity data lakes. These techniques can help uncover complex relationships, extract valuable intelligence from unstructured data sources, and identify sophisticated attack patterns that may evade traditional security measures.
Vulnerability Prediction and Risk Assessment
Beyond real-time threat detection and analysis, cybersecurity data lakes offer organizations a powerful platform for vulnerability prediction and risk assessment. By examining past incidents, organizations can uncover trends and commonalities in security breaches, weak points in their defenses, and recurring threats.
Cybersecurity data lakes store vast amounts of data spanning extended periods, which is a rich source of information for identifying recurring vulnerabilities or attack vectors. With techniques such as time-series analysis and pattern recognition, organizations can uncover historical vulnerability patterns through rigorous testing and use this knowledge to anticipate and mitigate future risks.
In fact, this is one of the reasons why the global pentesting market is expected to rise to a value of $5 billion by 2031, with more innovative approaches like blackbox pentesting to exploit hidden attack vectors and using AI for vulnerability assessment (VAS) to improve efficiency.
When combined with other vulnerability assessment methods like threat modeling and red team exercises, predictive modeling can also help organizations identify potential attack paths and attack surface areas and proactively implement defensive measures.
Security Insights and Reporting
Another benefit of cybersecurity data lakes is their ability to generate comprehensive security reports. By integrating data from sources like security information and event management systems, endpoint detection and response tools, and threat intelligence feeds, data lakes can provide a holistic view of an organization’s security framework.
These reports can cover a wide range of topics, such as incident summaries, vulnerability assessments, compliance audits, and risk analyses, enabling stakeholders to make informed decisions and prioritize security efforts effectively.
Implementation Challenges and Best Practices
While the benefits of cybersecurity data lakes are undeniable, their implementation comes with its own set of challenges, such as:
Data Privacy and Regulatory Compliance
Cybersecurity data lakes store vast amounts of sensitive data, including personal information, system logs, and network traffic data. Ensuring the privacy and protection of this data is not only an ethical obligation but also a legal requirement in many jurisdictions.
Organizations must implement robust data governance policies, access controls, and encryption mechanisms to safeguard sensitive information and comply with relevant data protection regulations like the GDPR and the California Consumer Privacy Act (CCPA).
Scalability and Performance Considerations
The sheer volume and velocity of data ingested into cybersecurity data lakes can quickly overwhelm traditional storage and processing systems. As an organization’s data footprint grows, ensuring scalability and maintaining optimal performance becomes a critical challenge.
To address this, organizations should consider implementing distributed architectures, leveraging cloud-based solutions, and employing techniques such as data partitioning and parallelization to scale horizontally and optimize performance.
Integration with Existing Security Infrastructure
Cybersecurity data lakes are not standalone solutions; they must seamlessly integrate with an organization’s existing security infrastructure, including SIEM systems, EDR tools, firewalls, and other security appliances.
This integration can be complex, requiring careful planning and execution to ensure compatibility, data consistency, and efficient data ingestion pipelines. Adopting standardized data formats, leveraging APIs, and implementing robust data management processes can help streamline this integration process.
Wrapping Up
By consolidating diverse data sources and taking advantage of advanced analytics and machine learning, data lakes allow organizations to detect threats proactively, mitigate risks effectively, and respond swiftly to incidents.
Organizations that embrace these advanced technologies can stay ahead of potential threats and ensure the integrity and security of their data in an increasingly digital world.