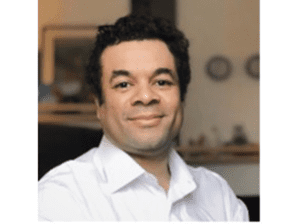
What are the biggest data strategy challenges facing you and your company?
If you are like most, the main reason for developing a data strategy is to be capable of supporting the growth strategy of each type of business in an exclusive way – to offer competitive resilience with balance and maturity to defend and attack.
In today’s business world, data is more than just a valuable resource – it is the backbone of intelligent operations and strategic decisions. However, many organizations often overlook the importance of adjusting their data strategies according to the specific context inherent to business growth. Whether through mergers and acquisitions (M&A), organic growth, strategic alliances, product or service development, expansion, or geographic diversification, each growth route brings with it unique challenges and distinct opportunities.
Each of these approaches brings unique implications for data strategy development and management and requires a unique, carefully architected data strategy to maximize value creation from the unique combination of proprietary data [1].
The effectiveness of a company’s data strategies is intrinsically linked to its ability to be aligned with its business context and growth strategies. Here are some important considerations for developing data integrations to enable analytics and capabilities with high potential to impact business decisions considering different types of organizations’ growth strategies, whether they sail in red or blue seas in search of competitive advantage [2]:
- Mergers and Acquisitions (M&A): In M&A operations, it is essential to carry out a detailed analysis of the systems and data assets of the companies involved. This allows you to identify redundancies, gaps, and opportunities for efficient data integration. Creating a unified data strategy helps ensure that the new entity can leverage the full potential of its combined data assets.
- Organic Growth: In the case of organic growth, companies must prioritize investments in infrastructure and data capabilities that can be scaled as the organization grows. This may include implementing flexible data platforms, adopting agile software development practices, and promoting a results-oriented data culture.
- Strategic Alliances: When forming strategic alliances, companies must establish clear data governance policies and data-sharing agreements to ensure the security and confidentiality of shared information. Additionally, collaboration around data analytics initiatives can generate valuable insights that benefit all partners involved.
- Product or service development: When developing new products or services, companies must adopt a data-driven approach from the beginning. This involves capturing and analyzing relevant market data, customer feedback, and performance metrics to inform the development process and ensure the successful launch of innovative products.
- Geographic expansion: During geographic expansion, companies must consider regional nuances regarding data regulation, customer preferences, and market conditions. This may require adaptations to your data strategy to ensure legal compliance, personalization of products and services, and the effectiveness of marketing and sales initiatives.
- Diversification: When diversifying into new sectors or market segments, companies must evaluate the interoperability of data systems and identify opportunities to share and leverage data resources across different areas of business. This allows you to maximize synergies and optimize the utilization of data assets across the organization.
Many top organizations in different sectors have implemented data strategies that match their business context well. For example:
- By growing organically, Amazon uses customer behavior data to tailor product suggestions and enhance user experience on its platform.
- IBM, through strategic partnerships with technology companies and cloud service providers, has expanded its offering of AI and data analytics solutions, leveraging diverse data sets and expertise.
- Oracle is a prominent example of a company that uses mergers and acquisitions strategies to boost its growth. Oracle has bought several technology companies over the years to increase its range of products and services. For instance, the purchase of Sun Microsystems in 2010 enhanced Oracle’s role in the hardware and software market, while purchases of companies such as BEA Systems, Peoplesoft, and Siebel Systems helped extend its enterprise software offering. Through these strategic purchases, Oracle has been able to reinforce its position as a leading player in the technology sector and offer complete solutions to its customers around the world. These purchases also have significant implications for Oracle’s data strategy, requiring systems and data integration to take advantage of acquired assets and provide additional value to customers.
- Tesla, by investing in research and development of autonomous driving technologies, collects and analyzes data from millions of vehicles on the road to continually improve its autonomous driving algorithms and increase driver safety.
A data strategy based on the business context must address the entire data lifecycle – from its integration to its use, and the ethical considerations that will arise, reinforcing that the strategy will direct the transformation of the organization and not the technologies [3].
When developing your data strategy, consider the following questions:
Creating value with data:
- For what business purposes will the data be used? This includes support for decision-making, personalization of products/services, and process optimization, among others.
- How can data be leveraged to drive company growth and achieve its strategic objectives?
Data acquisition:
- What types of data are needed to support the company’s growth strategies?
- Where will this data be obtained? This includes internal data, partner data, and third-party data, among others.
- What methods will be used to collect and acquire this data? This may involve the implementation of sensors, strategic partnerships, and acquisition of data from suppliers, among others.
Ethical and legal considerations:
- What are the ethical implications associated with collecting, using, and sharing data?
- How to ensure compliance with data privacy regulations such as GDPR and LGPD?
- What policies and procedures will be implemented to protect the privacy and security of customer and stakeholder data?
Data value and monetization:
- How can data be monetized to generate revenue for the company?
- What are the potential business models that can be explored based on the available data?
- How to maximize the value of data through advanced analytics and actionable insights?
Data integration and governance:
- How will data be integrated and consolidated into a single source of truth?
- What policies and processes will be implemented to ensure data quality, consistency, and integrity?
- Who will be responsible for data governance and management within the organization
Data lifecycle:
- How will data be managed throughout its lifecycle, from collection to storage?
- What criteria will be established to determine the relevance and ongoing usefulness of the data?
- How to guarantee availability and access to data, when necessary, while maintaining control over its security and privacy?
It is important to highlight that when data is integrated, it exerts network effects [4], because by integrating new data, new insights are possible and can enable the creation of new data products, new offerings for internal and external customers, new paths for disruptive innovations. New relationships created from data can be used to enrich, especially companies based on multifaceted platforms [5] who can experience the virtuosity brought by intentional data growth.
Certainly, coordinating the data strategy is not a simple effort and requires orchestrated work from the entire organization, which in recent years can count on the “chief data officer,” who normally represents data leadership in companies and in turn also actively leads revenue generation, sales discussions, and business beyond technology.
Considering the importance of a data strategy suited to the business context, the active participation of executives from all areas of the business (IT, sales, product development, legal, marketing) is highly relevant.
The present shows us that organizations are transforming into data and technology organizations and understanding how data strategy aligns with the business context is essential, as it allows organizations to make more sophisticated uses of data.
References
[1] Davenport, T. H., & Redman, T. C. (2020). Your Organization Needs a Proprietary Data Strategy. Harvard Business Review.
[2] Porter, M. E. (1985). Competitive advantage : creating and sustaining superior performance. Free Press ; Collier Macmillan.
[3] Kane, G. C., Palmer, D., Philips, A. N., Kiron, D., & Buckley, N. (2015). Strategy, Not Technology, Drives Digital Transformation. MIT Sloan Management Review and Deloitte University Press, 57181, 27.
[4] Becker, W. E., Shapiro, C., & Varian, H. R. (1999). Information Rules: A Strategic Guide to the Network Economy. The Journal of Economic Education, 30(2), 189.
[5] Hagiu, A., & Wright, J. (2015). Multi-Sided Platforms. Harvard Business Review, Working Paper, 15–037.