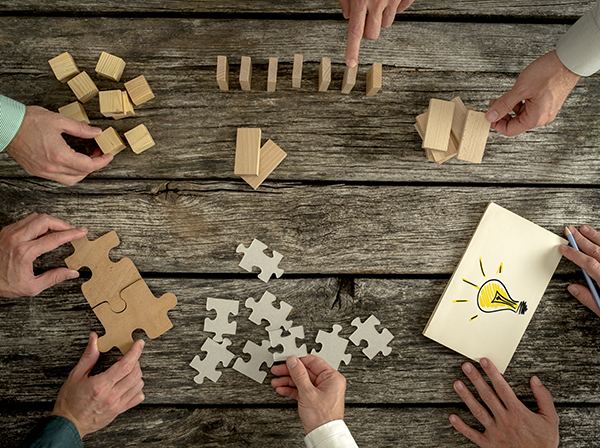
An organization can define its Data Quality goals and standards, and the steps needed to accomplish those goals, by creating a Data Quality framework. Creating it includes an assessment of the organization’s current Data Quality. A Data Quality framework can be described as an instruction manual for improving the quality of the data.
With a Data Quality framework, your business can define its Data Quality goals and standards as well as the actions needed to accomplish those goals.
Many large organizations are struggling to improve their Data Quality. They may have multiple data sources producing “almost” exact duplicate sets of data and creating consistency issues, or there may be anomalies impacting the data’s accuracy. Eliminating these concerns and attaining a high degree of Data Quality will improve decision-making and help in achieving long-term goals.
An effective Data Strategy framework can minimize the risks that low-quality data supports and improve the data being used for decision-making purposes.
As the Business Evolves
A start-up business may not initially have a need for organizing massive amounts of data (it doesn’t yet have massive amounts of data to organize), but a master data management (MDM) program at the start can be remarkably useful. Master data is the critical information needed for doing business accurately and efficiently. For example, the business’s master data contains, among other things, the correct addresses of the start-up’s new customers.
Master data must be accurate to be useful – the use of inaccurate master data would be self-destructive.
If the organization is doing business internationally, it may need to invest in a Data Governance (DG) program to deal with international laws and regulations. Additionally, a Data Governance program will manage the availability, integrity, and security of the business’s data. An effective DG program ensures that data is consistent and trustworthy and doesn’t get misused.
A well-designed DG program includes not only useful software, but policies and procedures for humans handling the organization’s data.
A Data Quality framework is normally developed and used when an organization has begun using data in complicated ways for research purposes. It is often used when a data lake is required for storage.
As an organization grows, it accumulates its own inhouse data, which, when analyzed, can be used to make the business’s internal processes more efficient. When massive amounts of data is gathered from outside sources for purposes of developing business intelligence, it is often stored in a data lake.
An Overview of Data Quality Frameworks
A Data Quality framework will help to maximize the business’s investments in data analytics by ensuring they are used properly, and provide accurate insights. However, to be successful, a Data Quality framework must be tailored to fit the organization’s needs. To be effective, the Data Quality framework must integrate with the Data Governance program’s policies.
As businesses continue to generate and collect more data than can actually be used, they need a Data Quality strategy to provide consistency. The lack of Data Quality framework can result in many challenges, including:
- Inconsistent data usage across the business: Different departments may interpret and utilize data in different ways, causing confusion and errors.
- Poor Data Quality: This can cause costly errors and result in the unnecessary expense of reworking of the data.
- A lack of transparency: Data that has been siloed, or stored incorrectly, may result in uninformed and poor decisions.
Preparing a Data Quality framework is time-consuming and may take two to three months.
Assessing the Organization’s Current Data Quality
An assessment of the organization’s current Data Quality is a good first step in developing a Data Quality framework. Data Quality assessments show where the data comes from, how it flows through the organization and is used, and the data’s quality. Additionally, the assessment identifies gaps in Data Quality, what type of errors the data has, why it has that level of quality, and how to fix it.
A more detailed assessment process can be found here. The basic steps for assessing the organization’s Data Quality are listed below:
- Begin with developing a list of Data Quality concerns that have been discovered over the last year.
- Spend time (a week or two) watching the flow of data. Look for questionable processes and the source of the problem.
- Share the discovered issues with other staff, ask for feedback, and include their suggestions in the assessment.
- Examine the list of Data Quality concerns and determine which are having the most impact on revenue. These should be considered high priorities.
- Reorganize the list of data concerns, with the priorities listed first.
- Establish parameters – what data will be examined during the assessment?
- Establish who uses the data, and determine their data usage behavior, both before and after completing the assessment. This will determine if they must make additional changes.
Creating the Data Quality Framework
Data Quality frameworks are fast becoming an important part of the Data Management puzzle. They support working with both external data from customers or suppliers (marketing projects, advertising campaigns, the customer experience) and internal data to streamline business processes.
The development of a Data Quality framework can be based on the steps listed below:
Understand the organization’s needs: This involves identifying the critical types of data that are used in making data-driven decisions. The data critical to making decisions appear in dashboards, reports, and other helpful decision-making tools. Understanding the organization’s needs also includes finding and correcting data flow issues.
Define the organization’s Data Quality goals: This usually involves working with Data Quality “dimensions” (e.g., accuracy, completeness, timeliness, consistency, and relevance), which are used to determine the quality of the data. Each dimension should be used in measuring the data’s quality.
Profile the data: Data profiling uses software to discover and investigate Data Quality issues, for example, data duplication, lack of consistency in the data, and a lack of accuracy or completeness. This process can be used to understand the nature and extent of the business’s Data Quality issues.
Become familiar with the DG and MDM programs: This should involve conversations with data stewards or members of the Data Governance committee. Discussions should include common complaints, suggestions for improvements, and software compatibility issues.
Develop policies for improving and maintaining Data Quality: The Data Governance program should already have a series of useful policies, which should be examined and adjusted to support the Data Quality framework.
Research and implement automated Data Quality processes: Automation is necessary for providing high quality data. Automation is significantly faster than humans and eliminates human error. Implementing Data Quality tools can automate the processes of checking and cleaning data.
Implement observability: Dashboards have become a highly functional way of providing a monitoring system on the flow of data. A Data Quality dashboard will track, analyze, and measure a variety of datasets over time. Additionally, these dashboards offer an overview of the organization’s long-term performance.
Develop a philosophy of updating the Data Quality framework regularly: Unfortunately, Data Quality is not a one-time effort. It requires continuous effort to review and improve the framework. Request continuous feedback from the stewards. The Data Quality framework is meant to be a living document that evolves and adapts to changing circumstances. It should be reviewed and updated regularly to align with business’s needs and goals.
Train staff: It is important to educate staff and management on both the importance of Data Quality to the organization and the new processes and changes that have been implemented. Creating a workplace culture that understands the value of Data Quality is necessary to ensure the Data Quality framework functions properly.
The Future of Data Quality Frameworks
Data Quality frameworks are becoming increasingly important for organizations wanting to develop clear policies and procedures for their use of the cloud, data lake storage, analytics, and data in general. As organizations rely more and more on data when making decisions, a Data Quality framework becomes increasingly important in providing accurate, high-quality data.
As the use of the cloud, data lakes, and new technologies increases, so too will the use of Data Quality frameworks.