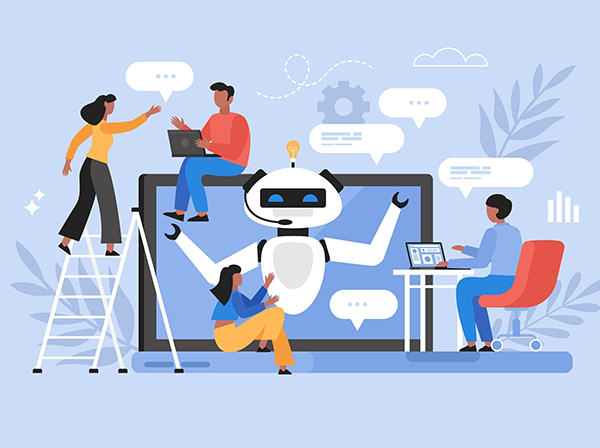
Artificial intelligence (AI) has witnessed remarkable progress in recent years, with one of its most notable achievements being the development of large language models (LLMs). These models have revolutionized the field of natural language processing (NLP), enabling machines to understand and generate human-like text at an unprecedented scale. LLMs are sophisticated AI systems trained on large text documents.
LLMs are trained to discover patterns and structures for comprehending context, semantics, and grammar intricately. By leveraging complex algorithms and deep learning (DL) techniques, these models can generate coherent paragraphs or even entire essays that appear indistinguishable from those written by humans.
The advancements in LLMs have significantly impacted various domains where human-machine interaction is crucial. From improving search engines’ accuracy to enhancing virtual assistants’ capabilities, these powerful models have demonstrated their potential for transforming how we communicate with technology.
However, the rise of LLMs has also raised important ethical concerns regarding misinformation or malicious use of generated content. In the case of LLMs, striking a balance between technological advancement and responsible AI usage is paramount.
The Role of LLMs in Language Modeling
Language modeling techniques form the backbone of LLMs, enabling remarkable advancements in text generation, text comprehension, and speech recognition. These models are trained to understand and predict human language patterns by learning from vast amounts of textual data.
Text generation is a key application of language models. By training on diverse sources such as books, articles, and online content, these models develop the ability to generate coherent and contextually relevant text.
LLMs enhance the human ability to extract meaningful information from written texts. Moreover, the application of LLMs extends to speech recognition technology. By leveraging a keen understanding of spoken words and their contextual relationships with other words within sentences or conversations, these models contribute to accurate transcription systems that convert spoken words into written text.
Text Classification and Semantic Understanding
Large language models also excel in text classification tasks. They can accurately categorize documents based on their content or sentiment analysis by effectively capturing nuanced semantic information from the text. This unique capability enables businesses to automate processes like content moderation, email filtering, or organizing vast document repositories.
Another remarkable aspect is their ability to grasp semantic understanding across different languages. By training on multilingual corpora, these models acquire a broader knowledge base that enables them to comprehend and generate text in multiple languages.
Contextual Information for Improved Performance
One key factor that contributes to the impressive performance of large language models is their ability to leverage contextual information.
They capture semantic nuances by recognizing the dependencies between words in a sentence and even across multiple sentences or paragraphs. This contextual awareness allows them to generate more contextually appropriate responses while minimizing ambiguity.
LLMs benefit from pre-training and fine-tuning techniques that refine their understanding of context-specific information. Pre-training involves exposing the model to a wide range of tasks with vast amounts of unlabeled data, enabling it to acquire general linguistic knowledge.
Fine-Tuning Large Language Models for Precision
Fine-tuning models for precision is a crucial process in adapting LLMs for specific tasks, such as language translation. While pre-trained models like GPT-3 possess a remarkable ability to generate coherent and contextually relevant text, fine-tuning ensures that these models can perform with even higher accuracy and proficiency in targeted applications.
The process of fine-tuning begins by selecting a pre-trained model that best aligns with the desired task. For example, if the objective is to translate text between languages, a model previously trained on diverse multilingual data might be chosen as the starting point. Next, the model is further refined by training it on domain-specific or task-specific datasets. During fine-tuning, the model’s parameters are adjusted through iterative optimization techniques. By exposing the model to labeled examples from the specific task at hand, it learns to make predictions that align more closely with ground truth.
This adaptation process allows the model to generalize its knowledge and improve its performance in areas where it initially lacked precision. Fine-tuning also enables customization of models based on factors such as vocabulary or style preferences. By adjusting some specific parameters during this process, practitioners can control how much influence pre-existing knowledge has over new tasks.
The Impact of NLP, ML, and DL on Large Language Models
NLP, ML, and DL form the backbone of large language models.
NLP is a subfield of computer science that focuses on enabling machines to understand and process human language. It involves various techniques such as tokenization, part-of-speech, and so on.
DL is a subfield of ML that employs artificial neural networks with multiple layers. These networks learn hierarchical representations of data by progressively extracting higher-level features from raw input.
DL has revolutionized NLP by enabling models like GPT-3 to handle complex language tasks. LLMs train on massive amounts of text data to learn the statistical properties of human language. They encode this knowledge into their parameters, allowing them to generate coherent responses or perform other tasks when given textual prompts.
Neural Networks and Transformer Models
Neural networks form the foundation of LLMs, enabling them to process vast amounts of text data. Neural networks consist of interconnected nodes units organized into layers, with each unit receiving inputs from the previous layer and producing an output signal that is passed on to the next layer. The strength or weight of these connections is adjusted through training, a process where the model learns to recognize patterns within the data.
Transformer models, a specific type of neural network architecture, have revolutionized language processing tasks. They introduced attention mechanisms that allow the model to focus on relevant information while processing sequences of words. Instead of relying solely on fixed-length context windows like previous models, transformers can capture large “textual contexts” by attending to all words in a sentence simultaneously.
The key innovation in transformers is self-attention, which enables each word representation to consider dependencies with other words in the sequence during both encoding and decoding stages. This attention mechanism allows for better understanding of contextual relationships between words and facilitates more accurate language generation and comprehension.
Large Language Model Applications: From Text Completion to Question Answering
OpenAI’s GPT-3 LLMs have garnered significant attention due to their remarkable ability to understand and generate human-like text. These models have found diverse applications, ranging from text completion tasks to more complex question-answering systems.
These models excel at predicting the most probable continuation for a given prompt, making them invaluable tools for content generation in various domains. Users can rely on these models to assist them in creating coherent and contextually appropriate content, whether that is writing poetry, coding, or drafting emails.
The GPT-3 LLMs have revolutionized question answering by providing highly accurate responses based on provided queries. By training on vast amounts of data from diverse sources, these models possess an extensive knowledge base that allows them to comprehend and answer questions across various topics. This has immense implications for fields such as customer support, education, and information retrieval.
Models like GPT-3 are pre-trained on large datasets from the internet, allowing them to learn grammar, facts, and even some reasoning abilities. Fine-tuning then allows these pre-trained models to be tailored for specific tasks or domains. Algorithmic advancements have also led to improvements in model performance and efficiency. Techniques like distillation enable smaller versions of large language models with reduced computational requirements while preserving most of their capabilities.
The Future of Large Language Models: Data Ethics
As language models continue to advance and evolve, it becomes crucial to address the ethical considerations that arise with their widespread adoption. While LLMs offer immense potential for various applications, there are several ethical concerns that need careful examination.
One key aspect is the potential for bias and discrimination within these models. Language models are trained on vast amounts of data from the internet, which can include biased information and perpetuate existing societal prejudices. This raises concerns about unintentionally reinforcing stereotypes or marginalizing certain groups.
Another important consideration is privacy and data security. Language models often require access to vast amounts of personal data to improve their performance. However, this raises questions about user consent, data storage practices, and potential misuse of sensitive information.
Additionally, accountability and transparency pose significant challenges in the future of language models. As they become more complex and sophisticated, it becomes difficult to understand how decisions are made within these systems. Ensuring transparency in algorithms’ decision-making processes is crucial to avoid any potential manipulation or bias.
Lastly, there is a concern regarding intellectual property rights and ownership over generated content by language models. As these models become more capable of producing creative works such as articles or music compositions autonomously, determining authorship and copyright regulations becomes increasingly complex.
Image used under license from Shutterstock.com