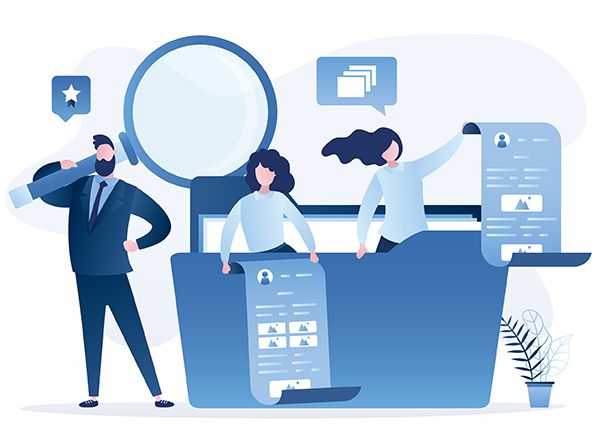
Data cataloging tools work with data catalogs to make them more efficient. Data catalogs typically come with tools included as part of the data catalog package. The tools included with data catalogs have been developed to support data quality, analytics, and compliance with data privacy regulations. Unfortunately, the number of independently sourced tools for data catalogs is essentially nonexistent.
Generally speaking, the independent tools described in various articles as supporting data catalogs are data analytics platforms, which use the data catalog as a tool.
In most articles titled “Data Cataloging Tools,” the topic ends up being about data catalogs, not the tools designed to supplement them. (Software developers take note: The sheer volume of searches suggests a need for data cataloging tools.)
Data catalogs are used to develop and store the detailed inventory of an organization’s data assets and are designed to help researchers locate useful data, as needed. They use metadata – a label using data to summarize and identify data files and assets – to collect, organize, and access the data, and to support a searchable inventory for the organization’s data.
The data catalog’s inventory provides researchers, analysts, and other data users with streamlined access to the organization’s data.
When the data catalog was first introduced, it was a simple, basic metadata management tool used by IT teams. With the development of big data research, data catalogs had to become more functional, flexible, and intelligent. Machine learning algorithms supported the development of these improvements.
A modern, well-designed data catalog should have machine learning capabilities, making research and data analysis quick and efficient. It should show users the available data assets, their location, and their relationships to other data assets and metadata.
These machine learning processes support metadata discovery tools, which help to keep the data catalog relevant and comprehensive.
Machine Learning Tools for Data Catalogs
The use of machine learning with data catalogs is having a significant impact on their efficiency. Machine learning (ML) is being used to augment modern data catalogs and to automate the use of metadata for research and data profiling (developing useful summaries of the data). The tools used by so-called machine learning data catalogs are typically a part of the package.
Machine learning – a fundamental part of artificial intelligence – uses algorithms to automatically make decisions when storing and locating data in the data catalog.
A machine learning data catalog tool uses advanced algorithms and techniques to support a variety of automated services. These catalogs will scan data and metadata automatically. They help in discovering data structures, relationships, and content.
Machine learning data catalogs will also streamline and automate data curation processes, including classification, data tagging, and the association of the business’s glossary terms to its technical data assets. They boost productivity and accelerate the completion of projects by automating common Data Management tasks.
A machine learning data catalog should include these features:
- Data classification: Data assets and files should be automatically classified and stored appropriately. This classification process should include automatically inspecting content for values and patterns within the data.
- Data discovery: This provides a way to identify, classify, and inventory an organization’s data across a variety of data landscapes, such as branch offices and the cloud. The process includes connecting different data sources, cleaning and prepping the data, and making it available throughout the organization. It also detects patterns and aberrations.
Machine learning data catalogs provide the automatic cataloging of data, with context, and in real time.
- Data tagging: This adds metadata to data files and data sets using key-value pairs, which provide context to the data. Data tagging makes the data easier to locate and work with. Data tagging is especially useful for research and analytics. It allows users to find data more efficiently by associating portions of information (for example, websites or photos) with tags or keywords.
- Data lineage: This is the automated process of tracking data as it changes, providing an understanding of the data’s source, the changes made, and the data’s destination within a data pipeline. Data lineage provides a record of the data throughout its history, including any transformations that may have occurred during ELT or ETL processes. The use of data lineage improves data quality.
- Data curation: This process involves collecting, cleaning, organizing, and labeling data. ML data catalogs will validate and organize the metadata using machine learning algorithms. Data curators frequently use the data catalog as a source of trustworthy information.
- Semantic inference: In 2001, Tim Berners-Lee (inventor of the world wide web), Ora Lassila, and James Hendler published an article in Scientific American introducing the concept of the Semantic Web, which in turn led to semantic inference. Semantic inference has recently been applied to data catalogs – and will continue to be developed.
Other automated services that should be available with the use of an ML data catalog are:
- Metadata extraction
- Tagging and classification of data
- Discovery of relationships among data assets
- Delivery of intelligent recommendations to researchers
- Profiling of data to assess its quality
- Associating business glossary terms with technical data assets
- Semantic searches
Data Cataloging Tools: What to Look For
Machine learning data catalogs are superior to earlier data catalog designs because they track data lineage and analyze how data is used internally. Tracking data lineage has become necessary for addressing privacy protection regulations (GDPR, CCPA). Additionally, they can process metadata from new and current data sets, tagging them per the organization’s rules.
Because ML data catalogs work in real time, they can assist in processing streaming data from the Internet of Things (IoT) and support real-time analytics.
Other issues to consider are:
- International legal and regulatory compliance: Currently, 107 countries have established regulations designed to protect personal data privacy. A data catalog can simplify complying with these regulations by profiling the business’s data assets, inferring (as in “semantics inference”) their relevance to regulations, and classifying and tagging data assets automatically.
- Easy integration with data assets: The data catalog needs to be able to connect with all the assets in the business. Additionally, it may be useful to find a data catalog that can be integrated with on-premises systems, the cloud, and hybrid systems.
- Artificial intelligence as a concern: Increasingly, businesses are relying on their Data Governance software to coordinate and use artificial intelligence. As part of a Data Governance program, some data catalogs can help in tagging and preparing data assets for optimal AI use and transparency.
The Benefits of Machine Learning Data Catalogs
When data researchers can access the data they need – without IT assistance – they can work more quickly and efficiently. In general, data catalogs provide an inventory of data files and assets that make it easy for nontechnical staff to locate data.
Machine learning data catalogs, however, provide a better understanding of the data through improved context – researchers can access detailed descriptions of the data, including the comments of other researchers. This can provide a better understanding of how the data is relevant, before reading it.
Other benefits machine learning data catalogs can provide for businesses are:
- Improved data quality improves decision-making
- Relationship metadata is shown, per knowledge graphs, and provides a 360-degree view of the data, establishes semantic relationships, and allows users to perform quick searches
- Provides data anomaly detection, identifying sensitive personal data that should not be shared, and flags risky data assets and aberrations
- Automates data integration, data quality, data preparation, and other Data Management activities. It also accelerates the development of business intelligence by automating data discovery, tagging, and collaboration
- ML-augmented data catalogs learn from users over time
Implementing the Data Catalog
Implementing a data catalog into a Data Governance system requires a considerable investment in time and software – an investment most organizations would prefer to only make once. Listed below are the required steps:
- The first step in selecting a data catalog is creating a list of what automated tasks the data catalog will be used for.
- The second step involves researching data catalogs that meet your needs, fit your budget, and are compatible with the organization’s Data Governance program and software. (If your organization does not currently have a Data Governance program, it would be worth investigating.) A data catalog should be compatible with your organization’s software and tools, including data quality rules and business glossaries.
- The third step deals with scheduling the installation, and then performing the installation.
The Future of Data Cataloging Tools
Data catalogs are rapidly evolving into a form of data intelligence platforms. Some predict the data catalog will become a centralized system of records for businesses.
Currently, data catalogs are limited to structured data, but over the next few years, they can be expected to support working with semi-structured and unstructured data. The data catalog will become the primary location for research.
A variety of data cataloging tools will be developed to work with data catalogs.
Machine learning data catalogs work with active metadata rather than passive metadata. Instead of simply collecting metadata and storing it in a passive data catalog, machine learning data catalogs will provide a two-way communications system, sending enriched metadata back to the source, and updating the appropriate files and systems.
Image used under license from Shutterstock.com