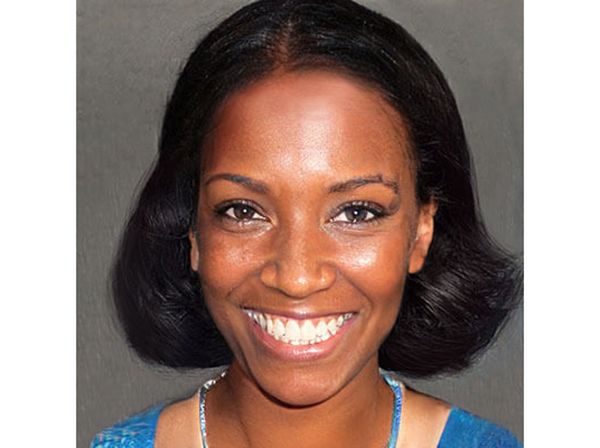
It’s no surprise that, in 2023, business enterprises want to become truly data-driven organizations. For many of these organizations, the path toward becoming more data-driven lies in the power of data lakehouses, which combine elements of data warehouse architecture with data lakes.
Data lakehouse architecture is particularly attractive to businesses that want to more rapidly digitize and invest more heavily in data-driven operations, and with good reason: Data lakehouses make it more straightforward for businesses to make their migration and data stack upgrade processes more efficient and less resource-intensive. Plus, data lakehouses offer multiple benefits such as higher data quality, better productivity, and the disintegration of enterprise-wide data silos.
To better explore businesses’ continuing investment in data lakehouse architecture, let’s take a look at what data lakehouses are and what role they may play in organizational data migration in the near future.
What Is a Data Lakehouse?
In order to define data lakehouses, let’s first quickly cover what data lakes are: They are, at their core, storehouses of data (in either raw or natural format). Data lakes store data from multiple sources – ranging from ML systems and dashboards to analytics systems and even mobile apps and social media – and it’s this multitude of technology sources that gave rise to data lakes in the first place; whereas conventional solutions such as data warehouses are best used for merely housing structured data, data lakes can subsume both unstructured and structured data.
The issue with data lakes, however, is their lack of necessary characteristics necessary to perform things such as artificial intelligence (AI) and data at scale.
They lack characteristics such as robust transactional features; governance, and ACID compliance.
That’s where data lake architecture comes into play – data lakehouses enable a merged data strategy, thanks to the marriage of data lakes and data warehouses to grant greater employee access and employment of data to ultimately inform business decisions.
In the eyes of plenty of businesses, data lakehouse architecture represents the next step forward when it comes to data stack upgrades that also enable agility and data flexibility. Successful adoption of data lakehouse models allows businesses to improve the quality of data, facilitate more productive collaboration, and disintegrate data silos that often plague larger enterprises.
Why Data Integration and Quality Benefit from Data Lakehouses
Now that you’ve got a good grasp on what data lakehouse architecture is and how it differs from data lakes, it’s important to dig into the biggest impacts data lakehouse architecture has on data integration and quality.
The construction of a modern data stack predicated on top of data lakehouses is essential to confronting pervasive issues relating to data integration and quality. Data lakehouse architecture relies on open-source tech and leverages data governance resources, and it addresses the daunting challenge of governing and securing large volumes of data in raw formats.
Basically, data lakes are placed on top of other lakes to maintain reliability and consistency when it comes to data; this is especially important in certain contexts such as open banking, where third-party developers are trusted to build applications for financial institutions and the security of financial data is paramount. Certain open-source storage frameworks that facilitate the creation of data lakehouse architectures also serve as reliable and open data storage and management layers for data lakes.
Data Lakehouses Disintegrate Legacy Systems and Silos
Most SaaS platforms relied on by modern businesses are, to their credit, able to provide a great deal of useful and insightful data. These massive volumes of data, when properly managed, can go a long way toward giving businesses a measurable and significant advantage over their competitors.
So, what stands in the way of businesses effectively leveraging this competitive advantage? Put simply, data silos: these data silos make it difficult for organizations to move away from legacy architectures and subsequently optimize their sets of data.
Issues often arise when organizations want to manage capabilities such as machine learning, artificial intelligence, and business intelligence in disparate data stacks; this contributes to greater levels of data complexity and further complicates the challenge of managing data scaling, data quality, and data integration.
Additionally, it isn’t feasible for organizations to manage ever-growing volumes of data with legacy tools – these tools can’t scale and subsequently cause teams to spend too little time gleaning useful insights from data that they have analyzed. It’s not uncommon for teams to spend a significant chunk of time during data analytics projects simply preparing data for analysis. To top it off, it’s no longer possible for teams to stand up a stack that’s dedicated to artificial intelligence workloads in light of how challenging it’s becoming to manage data replication across various platforms.
Paving the Way for Cost-Effective ML and AI
Businesses that are interested in handling and inexpensively storing raw data in various formats are typically those best suited to using data lakehouse technology. Lakehouse technology is also suitable for organizations interested in making their AI and ML use more cost-effective: Since data lakehouses combine the benefits of data lakes and warehouses, they can support various types of data workloads and types that AI and analytics tools need to ingest.
A common data repository, furthermore, creates a great level of control and visibility into data-rich environments, allowing data-driven enterprises to gain a competitive advantage in our modern hyperconnected, and rapidly digitizing world. Organizations also stand to enjoy sizable boosts in revenue as well as employee and customer retention thanks to their AI-driven investments.
One way for organizations to achieve AI-driven capabilities and tackle their data quality and data integration-related challenges is to embrace new data quality and governance tools as well as open-source solutions. To achieve this, it’s important that businesses either construct or invest in an infrastructure that provides a way for multiple teams to interact with data via a sole source, thereby allowing various data teams to work off identical information that isn’t outdated.
Conclusion
It’s clear that businesses striving to become more data-driven are interested in investing in data lakehouses to modernize their data stacks and subsequently grant their data teams more agility.
There are plenty of benefits that come with adopting lakehouse data models such as better data quality, productivity, and collaboration, as well as the disintegration of data silos. A modern data stack that sits on top of data lakehouses can also result in significant revenue increases for businesses as well as an uptick in customer and employee retention rates.