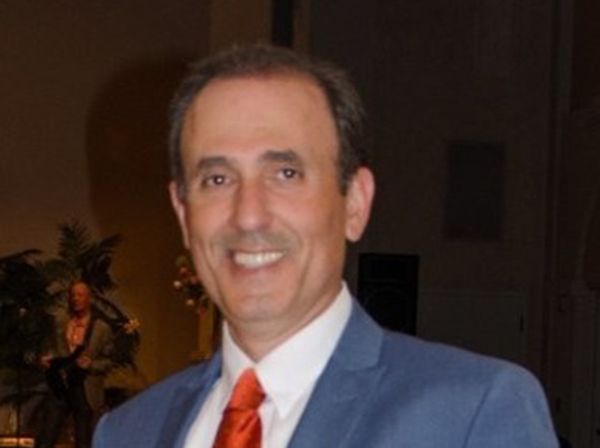
AI and data science have remained areas of focus in the last year and will continue to be top of mind for many tech companies in the coming year. Wearables, especially those with health monitoring capabilities, have also grown abundant as medical and consumer companies alike ramp up their offerings in response to increased demand. In addition, health care providers are becoming more open to the insights that wearables can offer from the rich data collected as a result of extended wear time. Here are the top trends in AI, data science, and wearables we should expect to see in 2023 and beyond, especially as they relate to health care.
New AI Approaches
There is no doubt that AI has become mainstream in many areas. In medicine, AI approaches are currently both developed and deployed at a rapid rate, fueled by the dearth of data that already exists from different modalities (genetic, genomic, images, EHR, etc.), as well as the continuous streams of data that are provided by wearables.
Most models today are based on supervised learning, where labels are combined with measurements to teach an algorithm to predict unseen data. However, it takes a lot of effort to create a labeled data set and as a result, usually only a subset of the data can be labeled – thus limiting the learning capacity of the current models.
In upcoming years, we can expect to see more AI approaches based on the use of self-supervised and generative AI algorithms be incorporated into product development.
Self-supervised learning can reduce the amount of required labeled data and by using a large unlabeled data set, a pre-trained model can be developed autonomously. Containing a rich set of learned features, this pre-trained model can be later fine-tuned for a specific supervised task, using a much smaller labeled set. The advantage of generative algorithms will be in the creation of synthetic data, especially when labels for the target domain are not abundant or other restrictions (e.g., privacy concerns) are present. If one, for example, wishes to create a more extensive and diverse set of training data in a new signal domain than those that are currently acquired through data collection, one can use generative algorithms to “translate” abundant signals from a relevant signal domain to the new domain. In both cases though, proper validation will be required to prove the validity of the algorithms, trained on such data, and the lack of any bias in their predictions.
Increasing Value of Data Science and De-Identified Data
Data has become a currency for many discoveries in today’s society, and we will continue to see its value grow in the next year. The integration of data sources – for a target set of the population and that covers an extensive set of features of interest – can have a profound impact on the generalizability and accuracy of AI algorithms. On the other hand, when these sets contain personal identifying information (PII) or protected health information (PHI) fields, integration of such sources in a training set are not possible without specialized procedures that eliminate specific fields and minimize the risk of identification of an individual. The problem is even more complex in medical applications, where patient data are protected by HIPAA.
In the next year, we expect to see commercial organizations seek to overcome this problem by using de-identification approaches that can link diverse data sets for the same individuals, owned and stored by different entities.
Tokenization is one such approach – it allows algorithm developers to gain access to diverse sets of data that are representative of the intended use population, which can then be used to develop and validate generalizable algorithms. Tokenization also creates an effective data search and exchange platform, where organizations can make available and find datasets of different modalities for the same patients, in a privacy-preserving manner. As real-world data becomes a major source for AI application development and validation, tokenization will play an increasingly bigger role.
Wearables and the Importance of Efficient Deep Learning Algorithms
The number of wearables in the market has accelerated and will only continue to increase. As these devices are miniaturized and come in a number of different forms (watches, rings, arm bands, etc.), a larger amount of data will be collected due to extended wear time. As a result, a major barrier will be the demand for battery life that allows for longer data collection and transmission of continuous signals.
This constraint emphasizes the need for more efficient algorithms on the devices, as well as the ability to compress the signal before transmission to the cloud for further analysis and storage. Developers will prioritize creating efficient deep learning-based algorithms, in order to provide an effective option to transmitting compressed signals that can be used to reconstruct important features of the original signal with high fidelity.
In health care, the availability of data collected over a long period of time, enabled by powerful compression techniques, will provide the ability for the development of cloud-based algorithms that not only diagnose existing health issues but can furthermore be used for risk stratification of the users. Such data would allow physicians to proactively follow up and treat patients at higher risk for the future onset of a disease. As acceptance of the clinical relevance of these devices increases in the medical field, the development of such risk stratification algorithms will require properly designed studies and prospective clinical validation efforts at a scale beyond that of current clinical trials. This will be another focus area in the next few years.
Summary
Innovations in AI and data science will lead to many more advancements in health care, especially when these innovations are applied in wearable technology. Proper validation in AI, tokenization of data, and increased clinical trials and validation of wearable data will provide a number of benefits to both providers and patients, including improvements to clinician workflows, care pathways, the patient experience, and overall health outcomes.