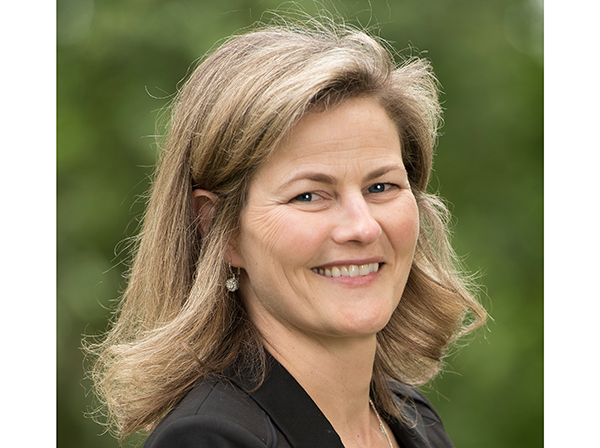
Data is the lifeblood of every company – even more so in the life science field, where this information is used to develop, approve, and launch new solutions to improve patient outcomes. However, correlating or connecting intelligent data points across the enterprise can be challenging. Doing so requires a robust enterprise information management (EIM) strategy developed specifically for the life science industry.
The following five critical elements must be considered when developing a purpose-built EIM system.
The Constantly Changing Data Landscape
The ongoing changes in the data landscape make developing a purpose-built enterprise information management system a complex process. With the widespread use of digital services transforming the terrain, life science companies are adopting a more data-intensive approach to business, supported by emerging technologies.
As consumer devices and applications become increasingly entrenched in everyday life, the amount of data generated multiplies exponentially. Currently, the world produces more than 80 zettabytes per year, which is projected to more than double by 2025.
In the life sciences environment, managing these quantities of data and extracting real-time insights requires sophisticated technology and comprehensive strategies to make it happen. Moreover, the acceleration in omnichannel engagement, the heightened cyber risks, and the burgeoning use of IoMT devices drive the requirement to span multiple information sources and pathways.
To optimize a company’s data investment, life science organizations need to develop a deep understanding of the data they possess, where it’s stored, and how they can use it to create value.
A Robust Data Governance Framework
A survey by Gartner identified the lack of a standardized approach to Data Governance as the single biggest barrier to achieving Data Management objectives. A robust Data Governance framework ensures that life science companies’ mission-critical data is usable, accessible, and protected. A purpose-built enterprise information management strategy requires a framework based on principles such as enterprise data security, standardization, anonymization, and master and reference data. The framework must define transparent processes, auditable and documented activities, and accountabilities for cross-functional and data-related decisions and controls.
Checks and balances are essential for all teams who manage the data and anyone who accesses or uses the information. A clear definition of the rights and responsibilities of all parties and established rules governing the collection and management of data will result in a comprehensive, sustainable enterprise information management strategy. Without an effective Data Governance framework, an enterprise information management framework can fail and fracture into an assortment of untracked data versions that pollute and impact the entire system.
Sound Data Quality Management Principles
An effective enterprise information management system must have a strong Data Management process encompassing its cloud migration strategies, data lakes, and data science capabilities. According to the EIM Institute, data is considered high quality if it fits the intended use and is correctly represented.
To achieve this, an enterprise information management’s governance framework must include procedures aimed at evaluating the quality of the data and ways to improve and maintain it across all datasets. Without adequate Data Quality management, companies end up relying on business intelligence that is incomplete, inaccurate, or out of date.
Detailed Data Acquisition Guidelines
Life science companies typically acquire their data in four different ways. They gather first-party data through customer interactions and touchpoints, such as new purchases, newsletter subscriptions, and social media. They can also transform or convert existing legacy records to a universal format that can be imported into the enterprise information management system. Finally, they share and exchange data with partners and suppliers or purchase datasets from vendors specializing in their target markets.
Data acquisition is a vital element of a purpose-built enterprise information management system. Establishing clear guidelines for how and where data is acquired, and who is responsible for the process, reduces the risk of duplicate and obsolete acquisitions.
An Encompassing Data Privacy Advisory
Data privacy is vastly important, and big news at present, with recent efforts regarding a bipartisan federal privacy law. For example, the American Data Privacy and Protection Act (ADPPA), released in June 2022 for review, follows the general framework of various state privacy laws and the European Union’s General Data Protection Regulation (GDPR).
Life science companies aiming to develop a purpose-built enterprise information management framework must ensure they meet the thresholds of current privacy legislation and consider whether any new bills or regulations, if passed, will impact their enterprise information management policies. Moreover, data privacy requirements vary between countries, so companies that serve global marketplaces need clear rules to comply with international, national, and regional requirements.
Since health records are now more valuable on the dark web, ensuring data privacy, access control and anonymization is critical.
The Takeaway
An effective enterprise information management system will deliver higher staff productivity and lower operational costs for life science companies. Advantages such as improved supply chain efficiency, enhanced customer service and loyalty, and more informed decision-making offer a real competitive edge.
With the deployment of a fit-for-purpose enterprise information management system, life science companies can maximize the value of their data, find business-driving insights, and benefit from the shift to more informed decision-making and greater digital transformation.