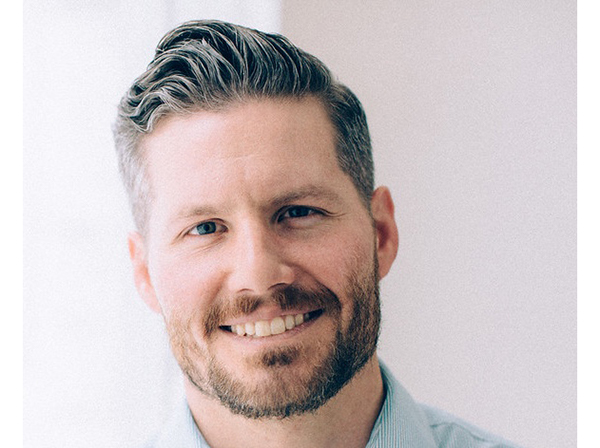
For business leaders to make informed decisions, they need high-quality data. Unfortunately, most organizations – across all industries – have Data Quality problems that are directly impacting their company’s performance.
Case in point: In a recent survey conducted by my company, practitioners were asked about the issues that plague their work, how much they trust their organization’s data, and how supportive their organizations are of Data Quality efforts. In a poll of mostly data engineers, 77% of respondents said they have Data Quality issues, and 91% said they were impacting their company’s performance.
Gartner estimates poor Data Quality costs organizations an average of $12.9 million by hindering their ability to make timely and confident decisions based on the data they have. It is a competitive advantage to maintain high Data Quality, according to the analyst.
The survey results clearly demonstrate the abundance of Data Quality problems among organizations, and how pervasive the resulting lack of data confidence is. When an organization has poor Data Quality, it lowers productivity and erodes confidence in the downstream artifacts derived from that data – like dashboards, machine learning models, and data applications.
The result is a disaster. Without a shared understanding of what your metrics are supposed to mean, you get what we call pipeline debt – a slowdown in your organization’s ability to make business decisions based on data, inefficiency and conflict between teams, and loss of confidence in the systems and workflows that rely on data.
Some key outcomes of the survey of 500 data practitioners (including data engineers, data analysts, and data scientists) include:
- Data practitioners cite various reasons for poor Data Quality in their organizations. These include lack of documentation (31%), lack of tooling (27%), and teams not understanding each other (22%).
- Fewer than half of respondents expressed high trust in their organization’s data, and 13% had low trust in Data Quality, which stemmed from broken apps or dashboards, decisions based on unreliable or bad data, teams having no shared understanding of metrics, and departments that were siloed or in conflict.
- Most organizations are supportive of Data Quality efforts, with 89% of respondents saying their leadership was supportive of Data Quality efforts, and 52% believing leadership regards Data Quality with high trust.
- Data validation is the norm: 75% of respondents said they validate data as a practice.
- Very few organizations are spared from Data Quality issues. Only 11% of respondents reported they did not have Data Quality issues.
In today’s world, data workflows and pipelines are complex, touching multiple stakeholders and changing through multiple processes. It has become a collaboration problem almost as much as it is a quality problem. There must be investment across an entire organization for Data Quality efforts to succeed. Data scientists, analysts, and engineers need support from executives to invest in the infrastructure and processes to improve an organization’s data quality, thereby enhancing business outcomes.
Efforts to improve Data Quality among survey respondents include having a Data Quality plan scoped and budgeted (22%), using a specific Data Quality tool (19%), checking data manually (14%), and building their own systems (15%). Respondents expressed a clear motivation to improve overall Data Quality.
Many organizations suffer from a lack of inertia. Data Quality can seem like such a mammoth problem – it’s hard even to get started. From my experience, though, organizations can have a meaningful impact by making small changes. Starting with one impactful data pipeline and following Data Quality best practices with just that one pipeline can have a measurable impact on business outcomes. This also begins the change in data culture and sets an organization up to improve over time.