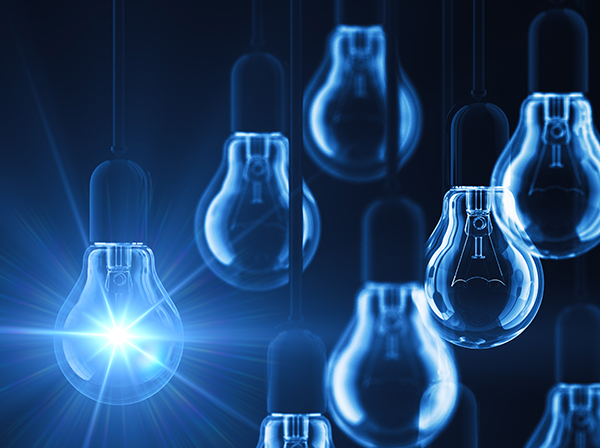
The hallmark of any successful Data Governance implementation is awareness. In the context of a large system integration project, we are talking about awareness of: 1) Data Quality expectations and metrics, 2) Enterprise Data Management plan, 3) Data Governance best practices, 4) data risk factors, 5) Data Governance framework, 6) data owners/data consumers, 7) Data Architecture principles, 8) data loss/confidential data requirements, 9) data inventory definition and locations, and 10) structured and unstructured data integration.
This list goes on and represents the knowledge needed to define and implement any project involving systems and data. Lack of this awareness from the very beginning inevitably leads to rework, project delays, and budget overruns. For the past 20 years, I have been involved in the proverbial lessons-learned meetings of project failures. Without exception, a root cause was that the team lacked awareness on the above points, and often went down an implementation path that required change and rework.
Based on our extensive experience in project triage and needed work we have been perfecting the art of Lean Governance. We considered ourselves specialists at second surgery, but the underlying problem across the industry is much worse than just fixing a warehouse or implementation. I was on the phone recently with a large insurance company undertaking a multi-year project. They acknowledged that Data Governance was a “separate thread that will start later.” When grilling them on the above questions, it was obvious this level of awareness had not been factored into planning and execution. The writing is already on the wall for that project’s chances of success and needed rework.
What is so special about Lean Governance that can help mitigate this serious project risk? First, I need to state that Lean Governance is not a synonym for Agile Governance, as I so often hear. We methodologists sure love our soap boxes. Simply put, Lean Governance is a way of looking at a business or data problem with the primary goal of driving out waste and risk at the least possible cost. Starting a project without the above information, and more, is an absolute waste and will result in reduced quality and cost overruns. Statistics will prove this point. In the 1980s, Toyota took serious market share from the Detroit Big 3 perfecting the concept of Lean Manufacturing. They did not consider lean as a synonym for agile. They did not chunk the mass production of cars into smaller units that could be more quickly delivered. On the contrary, they totally revamped the concept of manufacturing focusing on quality and customer needs first.
In a recent blog post, we equated data as raw inventory with the raw inventory seen in a manufacturing plant. A critical success factor for lean manufacturing was awareness of inventory. Clear labeling and knowledge storage locations. Putting stored parts next to the machines instead of three buildings over. The optimization of the flow of raw materials through the factory.
Lean Governance follows the same discipline. Isn’t classification, definition, and tagging another analogous to labeling inventory? Isn’t knowledge of data flows, data sources, lineage, and redundant copies analogous to inventory flows? The goal of IT is to deliver the finished good (e.g., system, report, model, etc.) to the customer with the required quality at the lowest cost and time. We start with data as raw inventory. As practitioners, let’s continue to learn from the masters that perfected this art on the manufacturing floor and turn the awareness to our own data factories.