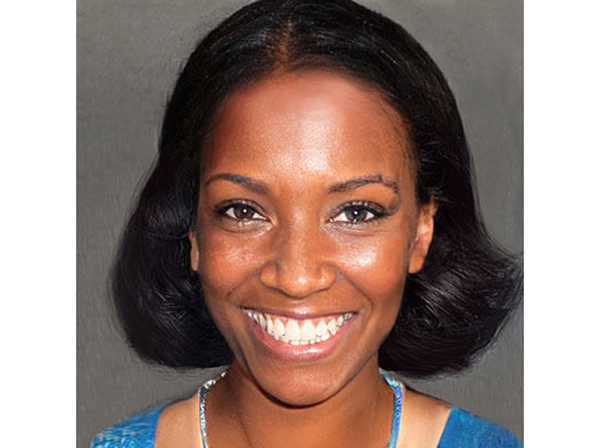
Data Science and statistics both benefit from transparency, openness to alternative interpretations of data, and acknowledging uncertainty. The adoption of transparency is further supported by important ethical considerations like communalism, universalism, disinterestedness, and organized skepticism.
Promoting transparency is possible through seven statistical procedures:
- Data visualization
- Quantifying inferential uncertainty
- Assessment of data preprocessing choices
- Reporting multiple models
- Involving multiple analysts
- Interpreting results modestly
- Sharing code and data
This article will discuss the benefits, limitations, and guidelines for adopting transparency in statistical practice. We’ll also look at some of the ways Data Science impacts business today.
What Are Data Science and Statistics?
Feel free to skip ahead if you’re already familiar with Data Science and statistics. Otherwise, this section will serve as a quick primer. Cassie Kozyrkov, Head of Decision Intelligence at Google, calls Data Science “the discipline of making data useful.” Statistics itself refers to collecting, organizing, interpreting, and presenting data.
Data Science is an interdisciplinary field that leverages fields like statistics, math, computer science, and information technology to make collected information useful. Today, Data Science is one of the leading industries because of the huge amount of data collected and leveraged by various corporations, governments, and people.
According to Glassdoor, data scientist ranks number 3 among the 50 best occupations in the U.S. In fact, many of the top jobs combine information technology training and mathematics, just like Data Science does. The importance of being able to process data will be key to success in the information age.
Next, let’s look at ways to promote transparency in Data Science and how that can be applied in the workforce today.
Visualizing Data
Let’s face it, an Excel spreadsheet of raw data is not the easiest thing to understand. This is why data scientists and analysts are so important. They help make sense of that data. One of the best ways to present information to demonstrate trends and outliers is by visualizing the data.
Data visualization isn’t just for interpreting data though. It can also help researchers explore data and build new theories and hypotheses. The key, however, is to leverage these visualizations for transparency. The power to show information can also be the power to mislead. For example, when comparing data sets through visualization, it’s important to use similar scales to prevent misleading data.
Data visualization becomes even more powerful with active models and static models too. Today, data scientists with computer science experience can build sophisticated models that dynamically respond to user inputs or show how data changes over time.
Quantifying Inferential Uncertainty
A common misconception about statistics is that it can give us certainty. However, statistics only describe what is probable. Transparency can be best achieved by conveying the level of uncertainty. By quantifying research inferences about uncertainty, a greater degree of trust can be achieved.
Some researchers have done studies of articles in physiology, the social sciences, and medicine. Their findings demonstrated that error bars, standard errors, and confidence intervals were not always presented in the research. In some cases, omitting these measures of uncertainty can have a dramatic impact on how the information is interpreted. Areas such as health care have stringent database compliance requirements to protect patient data. Patients could be further protected by including these measures, and researchers can convey their methodology and give readers insights into how to interpret their data.
Assessing Data Preprocessing Choices
Data scientists are often confronted with massive amounts of unorganized data. For example, data lakes are an increasingly common methodology for storing unorganized and organized data. They are highly scalable and allow you to run multiple types of analytics. However, once data has been processed, it’s important to assess and make clear how that data was handled before processing.
One issue with preprocessing choices is that they can lead researchers and data scientists to fall prey to their biases. As a result, the outcome of data can reflect only the most compelling results.
For example, a study by Steegen et al. reexamined another study that evaluated the connection between a woman’s relationship status (single vs. married) and her menstrual cycle and its impact on her religiosity. The study then applied various data preprocessing procedures. Ultimately, the study using a multiverse analysis found that the effect of fertility on religion was too sensitive to arbitrary choices and thus “too fragile to be taken seriously.”
Reporting Multiple Models
What’s the solution, then, to arbitrary preprocessing choices? Steegen recommends multiverse analysis in most cases and says that it is a way to avoid and reduce the problem of selective reporting: “To the extent their single data set is based on arbitrary processing choices, their statistical result is arbitrary.”
For example, imagine you’re a data scientist investigating your company’s supply chain. You may be inclined to exclude outlier data points in an analysis of your data. In a multiverse analysis scenario, you may include these data points. By including this information and investigating multiple models, your research becomes more robust.
Involving Multiple Analysts
Previously, I mentioned researcher bias as a force driving preprocessing choices. One way to mitigate bias is to involve multiple analysts. Researchers can decrease the impact of analyst-specific choices when multiple people analyze the same dataset.
The multiple analyst approach is also helpful because the more complex the data is, the more hands are needed to sort through it. One problem, however, is that the available manpower may limit the ability of multiple analysts to commit to a single project.
Artificial intelligence and cloud computing may offer a solution here. Blockchain is most frequently discussed in reference to buying and selling crypto on various exchanges. However, blockchain is starting to be used in Data Science too. Scientists could build multiple methodologies using neural networks and blockchain technology. This way, a single researcher could oversee a multiverse analysis by investigating multiple machine learning processes.
Interpreting Results Modestly
Data Science can be incredibly beneficial for decision-making. However, decision-making based on results that overstate their importance, replicability, and generalizability can be dangerous. Data scientists that give a modest account of outcomes enable readers to interpret and evaluate outcomes on their own merits.
One issue is that stronger language words like “amazing,” “ground-breaking,” “unprecedented,” and so on are more common. Textbooks also encourage authors to overclaim rather than remain modest with findings. By avoiding overstating claims, researchers ensure that the information conveyed stands on its own merit.
Sharing Data and Code
The importance of sharing data and code cannot be understated. Most importantly, sharing promotes reproducibility and allows others to perform sensitivity analyses. Other researchers can also validate the original work later on.
Data falsification and fraudulent data have become an increasingly common problem in academia. Sharing data enables other researchers to spot these problems. Just last year, Dan Ariely, the James B. Duke professor of psychology and behavioral economics, had two of his works come under scrutiny due to potential problems with his data. Had the data not been shared, this issue may never have been spotted.