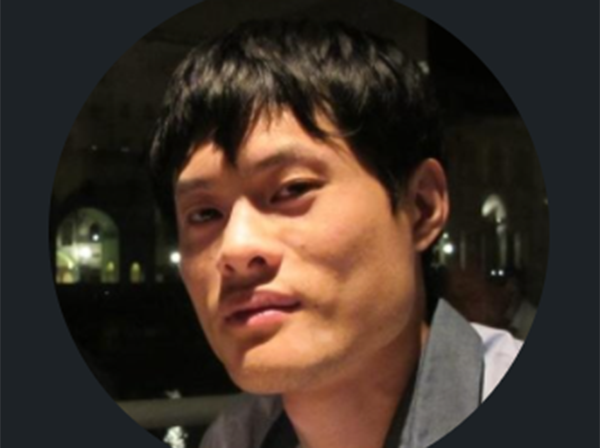
Imagine you’ve invited your boss over for a dinner party to try to show off your culinary skills (and perhaps get a promotion). The stakes are high, so you search the web and find the most revered chicken parmesan recipe around. At the grocery store, it is immediately clear that some ingredients are much more expensive than others. You think, “This is the best recipe around, so ingredient quality doesn’t matter!” and toss the cheapest cheese, chicken, and sauce you find into the cart. Fast-forward a few hours, and you open the oven to find a gray, shapeless mass that resembles anything but chicken parmesan. What happened?
Ridiculous as it may seem, many organizations treat their data operations the exact same way. Leaders with their fingers on the pulse of innovation understand the necessity for data in decision-making, but few properly vet what’s being put into their automated algorithms.
According to my company’s survey, 78% of executives struggle to make data-driven decisions, and 60% of executives don’t always trust the data they work with.
Moving forward, data quality will arguably be one of the most important investments leaders can make to ensure information-driven projects deliver on desirable business outcomes.
An Algorithm Is Only as Good as Its Data
Over the last few years, operations supported by big data and deep learning have gained so much exposure that leaders have started to overvalue large data quantities and undervalue data quality.
In 2018, Gartner reported that 85% of machine learning (ML) projects fail, predicting that the trend would continue through 2022. This is a direct result of poor data quality, which consequently leads to misinformed business decisions and a lack of creative collaboration between teams.
ML is made up of two core components: the model (or algorithm), and the data used to train the model. The former is a solvable problem, as most companies can download open-source ML libraries. When it comes to building out a production-grade ML system, however, data source and quality continue to be major issues.
Professionals often understand the benefit of data quality, but few have the time or resources to make it a priority.
When Data Ops Are Left to the Experts, Leaders Can Focus on Innovation
Gartner also reported that bad data can cost a company an average of $12.8 million per year. The antiquated, DIY process of internal data quality curation is unsustainable and leads to inconsistencies that produce poor results. These decisions negatively impact revenue and profitability, bring time-to-market to a standstill, erode customer trust, and significantly increase compliance risks.
With a data-first approach, leaders from every department can frequently communicate each others’ needs and expectations, and innovation is encouraged.
At energy-from-waste company Covanta, success is impossible without mature data quality practices. Operating the facilities is immensely complex, and the company must balance countless variables to ensure worker safety while generating clean electricity and maintaining maximum uptime.
Using the data supply chain management approach, the company established the Covanta Data Hub, where information is easy to find and readily consumable, and quality is assured. As a result, Covanta is able to see a wide range of data sources communicating in real-time, and extra cost was chopped. It took under four months to build out the data supply chain management solution, and in turn, Covanta is seeing 10% savings per year for maintenance activities alone.
For organizations to create and maintain a culture of collaboration, driven by quality data, success metrics must be defined and unacceptable practices – such as biases or unethical facets of datasets – must be universally agreed upon.
In an ideal data quality implementation process, experts meet with internal teams to understand needs and expectations, review datasets, and work to define the quality and monitoring rules that should be applied. Similar to the source of ingredients that determines your meal quality, the environment in which data is pulled is a central deciding factor in the success of a project.
Organizations that embrace data quality see massive improvements in cost savings and time-to-market. It gives business leaders a sustainable path towards healthier data and stress-free AI and ML practices without expensive tech or headcount investments. As data quality becomes a regular practice for teams, a holistic culture will develop where individuals can bring their own expertise and understanding of the problem.