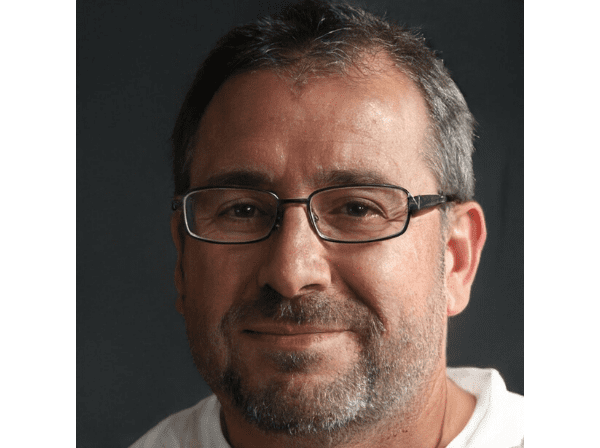
Click to learn more about author Bernard Brode.
Take a quick look at the history of big data, and one fact will immediately strike you: The ability to collect data has almost always been larger than our ability to process it. Processing power used to expand exponentially, but in recent years that growth has slowed. The same cannot be said of the volumes of data available, which continue to grow year after year.
The figures on this are startling. More data was generated between 2014 and 2015 than in the entire previous history of the human race, and that amount of data is projected to double every two years. By 2020, it was projected that our accumulated digital data would grow to around 44 zettabytes (or 44 trillion gigabytes) and to 180 trillion gigabytes by 2025. Despite this concentrated effort to acquire data, less than 3 percent of it has ever been analyzed.
Whatever the other big data trends of 2020, then, one is arguably more important than all the rest: the sheer amount of data available and the problems that will cause us. In this article, we’ll look at just a few.
Data Volumes Are Increasing Faster Than Ever
There are a few key reasons why data volumes continue to increase exponentially. One is simply that more and more people are conducting all their business and personal lives online. If you live in a relatively affluent part of the world (or, in fact, the USA), it can be easy to forget that the “internet revolution” is far from over yet. Internet penetration in the USA still lags behind other countries, and so there are plenty of people who have yet to come online. As they do so, they will be entering a world in which their every step is monitored. This is largely so that they can be targeted with ads, but it has also given rise to huge repositories of information on individual internet users.
The second major reason why data volumes continue to increase is the Internet of Things (IoT). A decade ago, the IoT was largely limited to primitive fitness trackers and medical applications. Now, a bewildering array of devices are designed to acquire data on their owners’ habits and send this data back to enormous data warehouses.
Where Are We Going to Store It?
For marketers, this increase in the amount of data available on the average consumer has undoubtedly been of huge benefit, and it has revolutionized the marketing industry. For network engineers, the explosion in data volumes has been less beneficial. That’s because all this data must be stored somewhere, and we may be approaching the limit of what is possible with traditional ways of doing so.
To see why, it’s worth getting an idea of just how much data we’re talking about. In its Data Age 2025 report for Seagate, IDC forecasts the global datasphere will reach 175 zettabytes by 2025. That’s right, we’re measuring in zettabytes now.
It would be an understatement to say that the systems currently used to store and manage this data are outdated. Until very recently, big data processing challenges were largely approached via the deployment of open-source ecosystems, such as Hadoop and NoSQL. However, these open-source technologies require manual configuration and troubleshooting, which can be rather complicated for most companies.
This was the primary reason that, around a decade ago, businesses started to migrate big data to the cloud. Since then, AWS, Microsoft Azure, and Google Cloud Platform have transformed the way big data is stored and processed. Before, when companies intended to run data-intensive apps, they needed to physically enlarge their own data centers. Now, with pay-as-you-go services, cloud infrastructure provides agility, scalability, and ease of use.
Big Data and Smart Data
As we’ve previously pointed out, though, the ability to store vast amounts of data does not, in itself, make the data useful. The crucial fact to remember here is that there is a difference between big data and smart data; the former is merely zettabytes of unstructured data, while the latter is useful intelligence.
Just as the need to store previously unheard-of amounts of data led to a revolution in the way that firms worked with IT, the ability to extract meaning from big data is likely to lead to fundamental changes in the way we interact with technology.
At the moment, most analysts believe that the only way we will be able to work with the huge datasets of the future will be via AI proxies. As the amount of data available begins to outstrip the ability of humanity to work with it, AIs are going to become a necessity.
In many ways, it’s strange that this shift has not occurred already. AI platforms have been around for a decade, and many are based on open-source architectures that theoretically allow any company to implement them. Unfortunately, a lack of expertise has held many back from doing so. Things are changing, though. AI vendors have started to build connectors to open-source AI and ML platforms and provide affordable solutions that do not require complex configurations. What’s more, commercial vendors offer the features open-source platforms currently lack, such as ML model management and reuse.
The Dangers
As this next transformation unfolds, however, we should take the time to learn from the last. The ethical implications of big data acquisition systems, which automatically collected and stored trillions of data points on billions of internet users, have only started to be recognized.
We should not make the same mistake with AI systems. There are some promising signs: Giants such as Google and IBM are already pushing for more transparency by building their machine learning models with technologies that monitor bias. However, in order to harness the potential of big data, we will need far more than advanced AIs and bigger storage centers. We will also need an ethical framework for when, why, and how this data can be used.