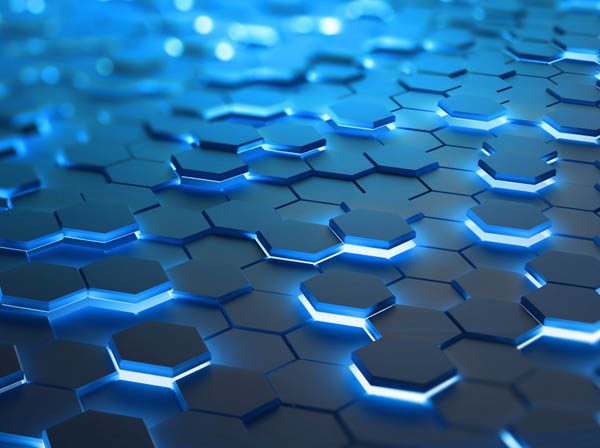
Peter Aiken disagrees with the popular idea that it’s impossible to put a dollar value on Data Architecture.
“It won’t be the right number, but it will be at least a dollar value on it, and if there’s money involved, people should be paying attention to it.” Aiken is an author, Professor of Information Systems, VCU and Founder of Anything Awesome. He spoke about Data Architecture and Data Strategy with attendees at the DATAVERSITY® Data Architecture Online Conference.
Data Architecture: Here Whether You Like it or Not
When clients ask Aiken’s company to develop a Data Architecture for their organization, he says they are asking the wrong question. “All organizations have architectures. The question is: do you understand your architecture?” If the existing architecture isn’t documented, he said, then it can’t be understood, and if it’s not understood, it cannot be useful to the organization. “Consequently, people say it is hard to put a dollar value on it.”
Data Architecture: A More Useful Definition
There are multiple definitions for Data Architecture, but most don’t pass the “elevator speech” test. As an alternative, Aiken offers these easy to understand and value-focused definitions:
- A common vocabulary expressing integrated requirements ensuring that data assets are stored, arranged, managed, and used in support of strategy
- A structure of data-based information assets, supporting strategy
- How we map out the common vocabulary expressing and ensuring that data assets are easily interchanged across the organization
- A structure of data-based information assets that supports strategy
“Most organizations have data assets that are not supportive of their strategies, which is why it’s hard to put a dollar value on information — because they don’t understand their Data Architectures.”
Organized Data has Value
Data that is better organized is more valuable than data that isn’t, he said.Attempting to find meaning in a random pile of pages is impossible without a table of contents, numbered pages, and an index. Likewise, organizations waste time and money when they have poor or non-existent Data Management practices, he said. “The major function of architecture is to get a handle on these things.” At the minimum, he said, 80 percent of the data in organizations is redundant, obsolete, or trivial (ROT). The process of reducing it entails separating the wheat from the chaff, but which data should be eliminated?
Strategy Prevents Disorganization
Typically, components are developed in response to organizational needs and are integrated into the Data Architecture. IT implements those requirements via a package or custom-developed software. Aiken says this process is missing a key piece: “Strategy is what helps you to prioritize all of the things that are relevant to your Data Architecture efforts.” If the architecture wasn’t originally designed to support current strategy, then it needs to adapt, he said.
Strategy: Don’t Just Plan — Do Something
Although most organizations think strategy is a complex thing, Aiken says that it’s not, and in fact, if strategy is too complex, it is simply not useful. “Strategy is about making sure that everybody keeps doing the same things, except for the few things that you want them to change.” Originally “strategy” was a military concept that described a battle plan, but Aiken said it’s better to think of it is as “A pattern, and a wave, and a stream of decisions.” Instead of focusing on the complexities of a planning process, recognize that the real importance of strategy is to do something.
Successful Strategy in Practice
Walmart has a simple business strategy: “Everyday low price.” When a Walmart employee makes a decision that supports an everyday low price for a customer, he said, they know they will never get criticism for it because they are putting Walmart’s business strategy into practice. “It’s a good strategy in that everybody knows it, understands it, and associates it with them. It’s a simple message and it makes sense.”
Hockey player Wayne Gretzky also has a simple strategy for success on the ice: He skates to where he thinks the puck will be. “Again, very simple stuff.” This strategy has allowed him to maintain his place as the highest scoring ice hockey professional of his day.
Not a Successful Strategy
Napoleon at Waterloo faced two armies, the British and the Prussians which, when combined, were bigger than his own, commented Aiken. He decided to use a “divide and conquer” strategy, assuming each army would retreat toward their food supplies when attacked, which would lead them to divide and move in opposite directions. He led his troops to the center point where both sides met, thinking that he could turn right and attack the Prussians with his entire army as they retreated, and then turn left and attack the British.
His strategy was two parts. The first part was to hit the armies hard in the center to divide them. The second part of his strategy was not so simple. First, all soldiers turn right and beat the Prussians. Then all soldiers turn left and beat the British, “and by the way, do all this while somebody is shooting at you,” Aiken said.
Data Strategy Provides Guidance
The Data Strategy then has to be the highest level of data guidance that’s available to organizations. Good strategy should provide direction when people are faced with a series of uncertainties. To show value, it has to be focused on activities that are specific, measurable achievements toward a goal. A strategy should also take into account where the organization is on its particular data journey, build on its strengths, and compensate for its current weaknesses.
Technology is not the Problem
New Vantage Partners’ Big Data and AI executive survey of industry leading corporations found that, in spite of increasing investments in Big Data and AI, the percentage of firms self-identifying as “data-driven” is declining. Aiken said that if firms truly expect to derive meaningful business benefits from their data, they must become much more serious and creative about addressing the “human side” of data. “Culture’s impact on this is absolutely astounding.”
Ninety-two percent of respondents identified people and process as challenges to becoming data-driven, compared to 81 percent in an earlier study. Only 7.5 percent saw technology as a challenge, down from 19 percent the previous year. Numbers are similar for business adoption of Big Data and AI initiatives: 95 percent cited cultural and organizational issues as challenges, with 5 percent citing technology.
Exorcising the Seven Deadly Data Sins
Aiken identified seven areas where people and process can be improved:
- Not understanding data-centric thinking: In the “Data Doctrine,” Aiken and colleagues say that leaders can increase organizational effectiveness by focusing on data as a central, shared resource (or better still) as your sole, non-depletable, non-degrading, durable strategic asset.
- A lack of qualified data leadership: “Call me crazy, but having somebody who knows what they’re doing at the top’s a pretty good idea.”
- Not implementing a robust program to develop shared data: Aiken emphasized that this should be a funded activity that operates continually rather than a project. He likens it to having a Human Resources group. “Nobody says, ‘Well I think we’ve hired our last employee today so we don’t need the HR group anymore.’”
- Not aligning the data program with IT projects: IT projects should be prioritized in a way that evolves existing Data Architecture toward more effectively supporting strategic needs.
- Failing to adequately manage expectations: Just like in HR, “being done” is not the goal, he said.
- Not sequencing Data Strategy implementation: After the foundation on a house is laid, building the walls and the roof makes it possible to work in the rain. As in building, he said, there are aspects of data that need to be sequenced that are not typically taught in school.
- Failing to address cultural and change management challenges: According to New Vantage Partners researchers, firms need to adopt a long-term approach, focusing on complex cultural challenges as a starting point.
Improving Data
Aiken suggested using Dr. Eli Goldratt’s Theory of Constraints, a systematic, repeatable process to identify, remove, and prevent obstacles to successfully using data in support of company goals. Then, over time, increase capacity and continue to improve so that focus evolves from reactive to proactive, he said.
- Identify the biggest constraint or block to supporting organizational strategy
- Attempt to quickly remove/correct the constraint operationally, without restructuring
- Subordinate all other non-constraints to ensure a singular focus
- Restructure to alleviate the constraint
- Repeat the process until data better supports the strategy
Understand Organizational Maturity
It’s important to keep in mind that one roadmap does not fit all businesses. Companies often develop a solution based on business needs alone, but Aiken says that’s the wrong approach because it leaves out the current state of the organization. He prescribes a different regime of mediation for organizations that are more mature on their data journey than for those that are less mature.
A Balanced Data Framework
He also suggests balancing higher profile business problem-solving with capability building processes.
Accomplishments that support the company mission and goals should be shared because those wins demonstrate value, but just as much effort should go into education and developing capability of new hires and existing staff. “If we don’t make up for the deficits of colleges and universities, and the gap that DATAVERSITY is filling via these conferences, then we will not be able to have any long-term success.”
Want to learn more about DATAVERSITY’s upcoming events? Check out our current lineup of online and face-to-face conferences here.

Here is the video of the Data Architecture Online Presentation:
Image used under license from Shutterstock.com