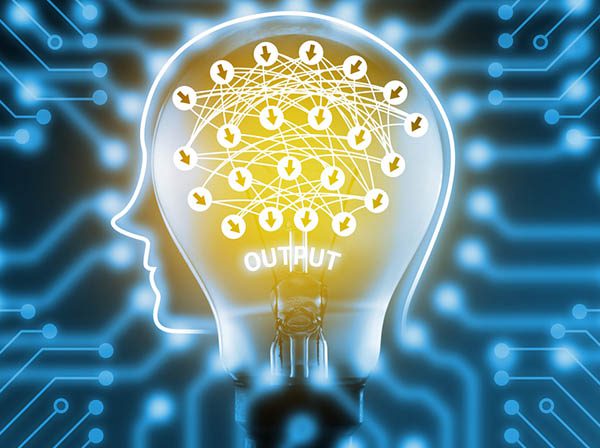
Emergent artificial intelligence (AI) technologies, especially the automated algorithms populating analytics platforms, are impacting and reshaping the world of business analytics. The underlying connections between traditional analytics processes and the disruptive technologies will make you cheer if you happen to be a data scientist or a business analyst — because your redefined role in the world of analytics promises a lot of exciting developments.
When AI technologies started germinating many years ago, the core idea was to teach machines to behave like humans. With machine learning (ML), the original core idea shifted to teaching machines to directly learn from training data created by humans. With deep learning (DL), another idea shift came when neural networks, emulating the human brain, took over the task of teaching machines.
Deep Learning Demystified offered this comparison of machine learning and deep learning:
“The ‘deep’ in deep learning refers to the number of hidden layers involved in the design. Unlike machine learning, which organizes and sends data through predefined algorithms, deep learning develops and uses basic algorithms to screen the data, and then trains the AI entity to ‘learn on its own’ by utilizing patterns, and ‘many’ layers of processing. A number of advancements taking place in the field of deep learning include: Deep learning and the use of neural networks currently offer the best solutions for many of the problems that come up in image recognition, speech recognition, and natural language processing.”
There are various intersection points between machine learning or deep learning and business analytics.
Advanced AI Technologies in Businesses Today
The State of Machine Learning in Business Today used an O’Reilly statistic to capture the essence of “AI technologies in the current business landscape.” O’Reilly finds that out of the 51 percent users of advanced ML technologies, 36 percent claim to be early adopters while 15 percent claim to be “sophisticated users.” The early adopters are busy incorporating automated ML in their business processes (embedded ML). The biggest point of contrast between ML and DL is that in ML, humans act as trainers for models, while in DL, neural networks emulating the human brain act as the teacher for training models.
AI, ML, and DL jointly attract substantial human interest in industry publications today. Learning continuously from streaming, real-time data is the new mantra in the post-IoT era, where “live intelligence” provides a clear competitive edge to businesses.
Intersection Point 1: Development of Training Data for Learning DL Algorithms
The deepest connection between ML or DL algorithms and business analytics is through the pre-designed business rules. In the AI-enabled analytics era, business analysts are no longer designing business rules; instead, they are developing appropriate training data for smart algorithms to learn those rules by themselves. Towards Data Science explains how the emergent AI technologies are impacting the professional roles of business analysts who are busy re-skilling to fit the new role of trainers.
Intersection Point 2: Accelerated Data Science
In accelerated Data Science, data analytics processes combine with ML or DL techniques to resolve complex problemsin the field of Data Science. Examples of accelerated Data Science include:
- In energy and utilities business, accelerated Data Science can be used to extract customer intelligence for enhanced product development, or for system vigilance to uncover risks or threats in a timely manner. The extracted customer insights enable accelerated decision making.
- In the healthcare industry, predictive analytics through accelerated Data Science can enable timely and improved healthcare decisions for a wide range of diseases.
- Data analytics, machine learning, and deep learning work collaboratively to optimize and deploy the trained neural networks in actual applications, while training deep neural networks through feature engineering.
In accelerated Data Science, the advanced AI technologies like DL add more sophisticated layers of data analysis to the existing analytics tools.
Intersection Point 3: Digital Ecosystems
The 2018 McKinsey Report Analytics Comes of Age talks about a digital ecosystem where several industry niches will converge to deliver single-window, customer-centric products and services. The core strength of this digital ecosystem is “multi-industry” solutions available via centralized digital access. In this scenario, once again, ML and DL algorithms will play key roles in enabling business rules for multi-vendor contracts, regulation of payments systems, and provisioning of common services like maintaining trading and trust relationship among ecosystem participants.
This ecosystem will reduce transaction costs, thus encouraging diverse businesses to contract out business activities. Recently, prompted by the growing need for customer-centric business models, leading businesses like Apple Pay, Alibaba, Amazon, Starbucks, GE, CBA B2B, and Ping An have all subscribed to the idea of “expanded digital services.”
And how are the global consumers benefitting from this ecosystem? They have gained access to a rich set of products and services across niches through a “seamless digital experience.”
Intersection Point 4: Deep Learning Analytics for Neural Networks
Deep Learning AI Based on Neural Networks Can Capture Trillions in Value reports that a recent McKinsey Global Institute (MGI) survey found DL-powered Neural Networks (many layers of learning) have the potential for a wide range of applications, the most significant of which is “creating between US$3.5 trillion and US$5.8 trillion in value annually.” This article also discusses recurrent neural networks and convolutional neural networks.
The MGI paper Notes from the AI Frontier: Insights from Hundreds of Use Cases offers an array of over 400 AI use cases across industry sectors and business functions. The paper states:
“Our use cases demonstrate just how powerful deep learning can be. On the other hand, deep learning networks can have seven to ten or more layers, with simulated neurons numbering into the millions…Advanced AI techniques could generate as much as 40 percent of the total potential value that all analytics techniques could provide.”
Intersection Point 5: Graph Analytics and Deep Learning
FUNL’s DeepInsight algorithm shows that DL techniques can help “analyze large-scale graph data.” Graph analysis, by its very nature, is suited for “in-memory” analysis. With the current growth rate of graph data, graph analysis and DL have mutual benefits.
Intersection Point 6: Use of Deep Learning in Big Data Analytics
Deep Learning in Big Data Analytics: A Comparative Study from Science Direct discusses the role of DL in big data analytics from a scholarly perspective. A strong use case for DL in big data analytics is found in Deep Neural Networks, Big Data, AI, and the Road to Autonomous Systems which traces the history and development of self-driving cars, as seen through the lens of the advanced software systems powering the central computer systems and numerous sensors in those cars.
Conclusion: Some Use Cases for DL-Powered Analytics
An example of McKinsey’s client servicing through advanced analytics is ACRE, the agricultural analytics center. This service outfit contains a team of data scientists, Software Engineers, and agricultural experts to apply advanced analytics (DL) to agriculture-related problems. The typical analytics services they provide are accurate weather predictions, accurate crop-pricing forecasts, worldwide cost curves for major crops, and geospatial analyses of local markets.
Another use case is chosen from the healthcare industry. The use of DL techniques for risk stratification in healthcare is explained in Deep Learning Bests Traditional Models in Risk Stratification. Healthcare risk stratification is “based on data collected through a multitude of sources such as medical history, health indicators, and the lifestyle of an adult.” It states the main purpose of risk stratification in healthcare is to tackle such issues as population-management challenges, custom healthcare services, risk-matching with available levels of care, and provisioning of healthcare practices with “value-based” outcomes.
Image used under license from Shutterstock.com