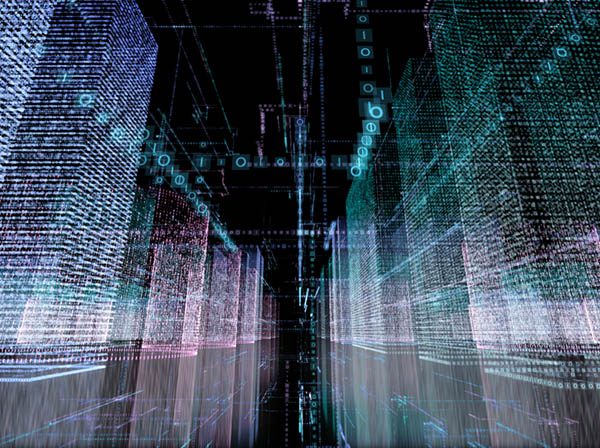
Data Architecture is tightly entwined with Data Strategy. It is the infrastructure to realize Data Strategy goals; the blueprint for managing data. It’s a big tent: ETL, Big Data, cloud, data virtualization, DataOps, governance, analytics, and anything else related to describing, organizing, integrating, sharing, and governing data.
A look at many topics and discussions going on throughout the internet, as well as those covered at the DATAVERSITY® Data Architecture Summit, gives insight into many of the facets of a modern Data Architecture. The topics are a look at how companies will be tackling Data Architecture this year – and likely for many years to come:
- As a key component of a modern data ecosystem, next-generation data warehouses will have to remain true to proven practices of enterprise data integration while interoperating with data lakes, master data repositories, and analytic sandboxes in a fast, scalable and agile fashion.
- Data engineering will have to be rethought as a new era of data management begins marked by graph technologies – the fastest growing segment of the AI market.
- Developing a long-lasting enterprise model will require aggressive research into clues from legacy systems, more probing interviews of SMEs, and recognition of the external forces driving change in business architecture.
- A structured engineering culture must give way to that of the creative and innovative mindset that characterizes a true data-driven company.
- Data practitioners must become adept at creating Data Architectures that provide the necessary security and privacy for modern applications as advances in machine learning and other data-hungry systems continue.
- The trend of using multiple cloud vendors means organizations must focus on planning integration between the two platforms as well as integration with on-premise data.
- Data Governance initiatives often fall down in the last mile, and preventing that will depend upon a well-integrated information hub that is complementary to the organization’s operations.
- Self-service analytics implementations and business intelligence still aren’t pervasive throughout the business and they won’t be until there is a free flow of information. That involves removing bottlenecks, eliminating non-value-added tasks in the information value chain, and providing tools and training to all stakeholders to ultimately provide intelligence to the right individuals at the right time.
- As part of diving into DataOps, companies should apply the DevOps techniques from software development that they know in order to create an agile analytics operations environment, including how to add tests, modularize and containerize, do branching and merging, and more.
DataOps Gains Steam
Let’s start with that last point. Interest in DataOps has surged since Gartner named DataOps to its Data Management Hype Cycle about a year ago, says Chris Bergh, CEO of DataOps vender DataKitchen. More than 7,000 people have signed the DataOps Manifesto, which lays out the principles of DataOps as a better way to develop and deliver analytics.
“The DataOps view that analytics is a combination of software development and manufacturing operations seems to have struck a chord within the data industry,” Bergh says.
DataOps presents a sea-change in the capabilities and effectiveness of analytics teams, Bergh adds. A 451 Research report in February found that over the next 12 months, 86% of respondents planned to increase investment in DataOps strategies and platforms. This year likely will see that percentage rise, given that 92% of the respondents to the survey expected this strategy to have a positive impact on their organization’s success.
DataKitchen and Eckerson Research have research which showed that companies continue to struggle with analytics. Too many data errors negatively impact the productivity of the analytics team, affecting the entire workflow of analytics development, Bergh points out. Thirty percent of respondents reported more than eleven errors per month. Additionally, 75% of respondents said that it takes days, weeks, or months to create a development environment – a process that should take minutes, the report says.
That wait time prevents the data analytics team from even beginning to work on the critical analytics that the organization has requested. “This means that their time-to-value is much slower than it should be,” Bergh says. “We see DataOps as being at the heart of data pipeline modernization,” orchestrating data flowing into Data Lakes, marts, warehouses, and ultimately to analytics.
Eckerson has said that evolving practices and processes for data pipeline development and deployment, analytic model development and deployment, and operation of pipelines and models should be part of a business’ Data Strategy – and 2020 may see that happen.
Go-Forward Data Governance
Donna Burbank, managing director at Global Data Strategy, notes that business users are becoming hands-on with BI, analytics, and even data preparation:
“So, Metadata Management needs to become more prominent and accessible so that data definitions and lineage are clearly understood. Data Governance is a critical best practice to align business users with IT staff and to ensure that the proper guardrails are in place for sensitive and/or critical data.”
A trend Burbank sees going forward is that data analytics will be less about volume and more about value. “Many organizations are looking less towards big data initiatives and are instead focused on identifying the critical data areas that will offer the highest value and ensuring the proper governance, maintenance, and publication of those critical data assets,” she says.
The Data Governance of the future, according to Irene Polikoff, co-founder and CEO of TopQuadrant, puts knowledge graphs in the center of everything. They provide dynamically accessible, flexible, and extensible business models for the enterprise.
“The complexity and variability of enterprise infrastructures are only growing,” she says. “This means that Data Governance must become more of a reality within enterprises. To achieve it, engineers need to be able to build governance into their systems from the start, just as they do with key aspects of security, access control, logging, and analytics.”
As far as Data Governance goes, companies are getting serious about GDPR, according to Semantic Arts president Dave McComb. They’ll be carrying that attitude over to the California Consumer Privacy Act (CCPA), which is coming down the pike this year.
“Profiling data will become essential, as will compliance databases that connect regulations directly to policy, procedure, process, and data,” he says.
Talking Big Data and IoT
When it comes to big data and data streaming, particularly in areas such as manufacturing and Industry 4.0. initiatives, a common use case that is expanding a great deal is the Internet of Things (IoT), Burbank says.
In 2020, as IoT comes online, says McComb, businesses need to make the switch from data at rest in a database to data in motion on the wire.
“We will have architectures with serverless computing and stream processing so that, in some cases, the data will never persist,” he says. “There is marginal value in raw sensor data that didn’t trip any threshold and we now have devices capable of creating data at rates faster than any storage medium can persist.”
Digital Transformation Keeps on Going
Digital transformation initiatives are ever present, and Customer Experience (CX) will remain center stage in most cases, says McComb. But the issue is by no means resolved yet. “Most firms are struggling to get even to a 360-degree view, let alone a seamless experience,” McComb says. “Cataloging all your data and beginning the process of semantically integrating customer data is table stakes for digital transformation.”
In 2020, it’s time for companies to recognize that digital transformation is as much a business transformation as a technical one, marrying Data Architectures with business strategy.
“Too often, organizations simply mimic brick-and-mortar approaches online, which is a lost opportunity for innovation,” notes Burbank. “Integrating digital transformation with enterprise architecture (for example, business process models), design thinking approaches (customer journey maps, for instance), and data architecture (like platforms and Data Governance) is critical to develop a successful digital transformation.”
The Career of the Data Architect
Given the progression of so many facets of Data Architecture, businesses will be keen on hiring data engineers in the new year. “Their job is to try to make the 70% of the time that data scientists spend data wrangling more like 30%,” says McComb. Tools like data.world – which helps companies use data catalogs to lay the foundation for CCPA – and eccenca – a Data Management solution for driving automation and rationalization for data integration, analytics, and data-driven processes – may continue on an upward trajectory to give data engineers the raw materials and tools they need.
“Everyone is moving to the cloud and hoping it will change their economics. It’s changing things from CapEx to OpEx, but for many, the major costs of integration and conversion are not changing,” McComb states.
It’s time for data architects to get uncomfortable, recommends Burbank. With the industry changing so rapidly, it’s easy to remain in silos – but don’t do it. She says, “I’d suggest that any data architect should spend the time to evaluate one new technology in 2020 that is outside of their comfort zone.”
Image used under license from Shutterstock.com