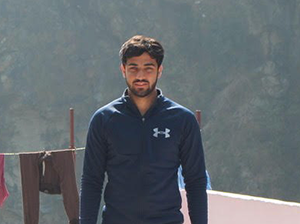
Click here to learn more about Harsh Arora.
Risk stratification in healthcare is defined as the process of assigning a risk status to all patients in a practice. The risk status is based on data collected through a multitude of sources such as medical history, health indicators, and the lifestyle of an adult or paediatric population. The objectives of stratifying risk include addressing population management challenges, individualizing treatment plans to lower risks, matching risk with levels of care, and aligning the practice with value-based care approaches.
Deep learning (DL) is an artificial intelligence (AI) function that imitates the mechanisms of a human brain in processing data and creating patterns to assist in decision making. It is also known as deep neural learning or deep neural network. Deep learning is a subset of machine learning (ML) that has networks capable of unsupervised learning from unstructured data. DL has been evolving with the digital era, which has brought about an explosion of data in all forms. The large amounts of data, known now simply as big data, is drawn from sources like search engines, social media, e-commerce platforms, etc.
AI, ML and DL all have gained a lot of attention for quite some time now and are all a part of the Fourth Industrial Revolution. These technologies are greatly transforming industries such as retail, manufacturing, finance, travel, etc. Healthcare is surely one such industry where you will find plenty of use cases for these technologies. As a result, DL in healthcare has offered path-breaking applications. They are able to gather massive volumes of data such as medical reports, patients’ records, and insurance records, and apply neural networks to provide the best outcomes.
Recently, a study published by the American Journal of Managed Care revealed that by leveraging deep learning and medical claims data, a predictive model was able to outclass traditional tools in pediatric patient risk stratification for hospitalizations. Current risk stratification models rely on groups of diagnosis codes developed in the early 2000s. While previous studies have tested the validation of the predictive ability of these models, research has mostly focused on adult patients.
Researchers also noted that most existing risk adjustment methodologies were created using a standard population and were therefore optimized with greater emphasis on adults. However, the potential attached to the pediatric populations was certainly constricted because of this. Due to the persistence of healthcare costs among certain groups of high-cost children, pediatric risk models benefit from including patient-reported measures of health status.
This team of researchers had set out to build a predictive risk model for pediatric patients using DL with clinical and financial data. The researchers collected data from 2014 and 2015 and developed a DL model that would predict a pediatric patient’s risk of hospitalization in 2016. The next step was to compare the model’s performance to that of traditional risk models. They also calculated costs for patients in the top 1 percent and 5 percent of hospitalization risk recognized by both models. The results were very clear and showed the DL model performed best compared to all the other models, with an area under the curve of 75.1 percent. The top 1 percent of patients selected by the DL model had a combined cost that was $5 million higher than the groups recognized by other predictive models. Also, in terms of accuracy, once again the DL model was in the lead. When it came to selecting the top 10 percent of high-risk patients, the DL model correctly identified 45.2 percent of all those who were hospitalized. The other models had predicted it at 39.2 percent and 41.6 percent respectively. These results conclusively demonstrate that DL and AI can help improve risk stratification.
This DL model, though in its preliminary phase, may enable population risk stratification sans the large investment of human capital, which is very significant in other modelling techniques. Other benefits associated with DL models include eliminating the need for domain knowledge and provider expertise to sort related medical claims into clusters. DL models also require far less time for results than other models, which human experts need years to polish and develop. This is of course only one of the use cases where such models have proven to be exceptionally advanced. In fact, the capacity to improve diagnostic prediction of a cluster of patients may also lead to better assessment of provider performance.
Although DL analytic approaches are gradually snowballing, the application of such models in risk stratification has been sluggish. Fortunately, there are some progressive care systems that understand the significance of this future tech and continue to support the research that demonstrates their value addition. In order for us to see a more rapid adoption of these innovative methods, several users and their perspectives will have to be taken into consideration. The biggest challenge attached to these technologies is the stigma and the ignorance in the mind of the public. For it to be more widely used and show its true potential, we as industry experts should not only learn about the benefits attached but also deep dive into the research that is being conducted.
We have seen across industries the impact that these technologies have had. They are not only able to give better results but also are able to reduce costs for organizations in the process. In an industry like healthcare, accuracy and cost effectiveness are certainly factors that are significant to not only the organizations but also to the patients. In conclusion, the DL model outperforms the traditional risk models in the area of hospitalization prediction. Therefore, DL may improve the ability of managed care firms to perform predictive modelling of financial risk and to, improve the accuracy of risk stratification for health management activities.