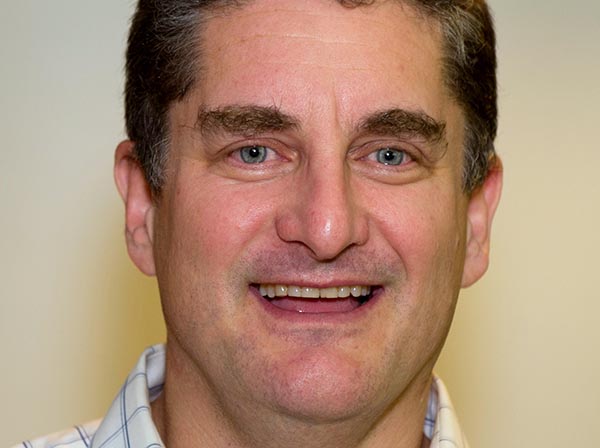
Click to learn more about author Sean Martin.
Machine learning and artificial intelligence’s preeminence is the natural outcome of the post Big Data landscape. Characteristics of this new reality have far surpassed the traditional “three v’s” of Big Data. Higher volumes of data now include greater distributions of data. Increased velocities are now real-time. Data’s variation is reflected in the varying tools, techniques, and technologies supporting them.
These demands have made the data fabric architecture a de facto requirement for deploying these cognitive technologies and ensuring them a steady supply of clean, integrated data. It’s crowned by the knowledge graph infrastructure that makes this complicated, decentralized architecture work by equipping the enterprise with:
- Integration of the entire stack of tools, technologies, and especially data utilized.
- Data discovery of which data’s available for which purpose.
- Machine learning practicalities of leveraging high-dimensional data that improve predictions yet make feature engineering more complicated.
Thus, this data infrastructure is the perfect playground for profiling, transforming, and preparing data for AI systems predicated on machine learning.
Relationships and Data Discovery
The nucleus of the infrastructure upholding data fabrics is the knowledge graph environment that specializes in identifying relationships among data. The assortment of use cases buttressed by graph technologies includes delineating and traversing relationships, linking metadata, detecting patterns, real-time analytics, and much more. Consequently, knowledge graphs enable heightened data discovery to inform machine learning with the most appropriate data. They’re critical for understanding connections between datasets and business problems. They are also a source of potentially predictive signals.
Blending and Integrating
Knowledge graphs are so pertinent for coalescing the variety data fabrics encompass because they’re based on methods that harmonize data with semantic standards. The resulting integrations are remarkable. Graph data models can describe any data variation, blending and linking multi-sourced, multi-dimensional instance data along with its describing metadata and context. These models are based on business terminology of standardized vocabularies and taxonomies. Therefore, regardless of which sources, structures, or formats data originate from, they all impeccably blend in a semantic graph that is an ideal starting point for feature engineering. For example, financial analysts can readily integrate news reports, spreadsheets, social media profiles, and more to identify the most profitable investment predictions for customers.
Machine Learning Essentials
Once organizations know what data’s available, cleaned, and integrated, they can prepare machine learning models with feature engineering. This process involves further transforming data so they’re suitable for machine learning algorithms. Relational technologies are far less appropriate for wrangling and transforming the multi-dimensional data yielding effective machine learning features. Graphs involve less time and effort for extracting data for specific use cases. In health care, a single patient’s data might be strewn throughout dozens of interrelated tables. Graph approaches handily link and transform this info to produce feature-sets that can make predictions about whether a patient is likely to suffer a disease, for example. They support more data for a richer understanding of machine learning model features and are a single place to profile and transform data to prepare for this technology. Increasingly, we are seeing the machine learning workflow steps (profiling, transformation and embeddings, training, etc.) take place directly against the data in the graph without any need to extract it into a downstream pipeline, a huge saving in time.
The Bottom Line
Realistically, organizations can do all of these processes in other environments. However, they’ll spend far more time and money doing so, with greater overheads leading to decreased ROIs and missed opportunities. Conversely, the graph approach is purpose-built for these tasks and, subsequently, excels at them.