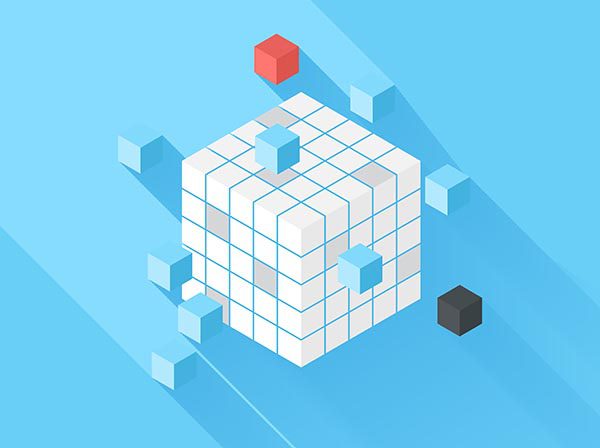
Given the high volume of erroneous data floating across the enterprise, it is increasingly difficult for industry operators to make business decisions or plan any course of action based on such poor-quality data. Moreover, what’s the point of applying advanced analytics or BI technologies on data that is flawed?
A whitepaper titled Getting Ahead of the Game: Proactive Data Governance reviews the role of Data Quality (DQ) in an enterprise Data Governance (DG) framework, and also provides a critical analysis of the close connection between the two concepts. According to Gartner, about 40 percent of enterprise data is “either inaccurate, incomplete, or unavailable,” which leads to an estimated annual loss of approximately $14 million. What are organizations to do?
Data Quality and Data Governance: What’s the Connection?
Although Data Quality and Data Governance are often used interchangeably, they are very different, both in theory and practice. While Data Quality Management at an enterprise happens both at the front (incoming data pipelines) and back ends (databases, servers), the whole process is defined, structured, and implemented through a well-designed framework. This framework for managing enterprise data may be thought of as Data Governance framework, where rules and policies related to data ownership, data processes, and data technologies used in the framework are clearly defined. So, Data Governance provides the framework for managing Data Quality.
InCountry Launches Data Residency-As-A-Service for Multinational Organizations discusses a one-stop regulatory solution for all multinational or country-specific business operators grappling with newly emerging compliance laws and policies. This solution enables businesses to store data locally, thus avoiding across-the-border compliance issues.
Use of DQ and DG Strategies in the Financial Services
Another Data Governance use case is sharply visible in financial services. In the digital-banking industry, DQ and DG have been exploited to transform entire business models. The banks that judiciously leveraged data platforms to reduce risks, streamline expenses, and boost revenues have impacted their bottom lines by 15 to 20 percent. The financial services industry leadership has now realized that a strong Data Strategy, which includes Data Quality and Data Governance, is the answer to developing efficient business models.
The major drivers of this transformation are, of course, explosive volume of data, dramatically reduced data-storage facilities, and high-speed processing. The increased focus on regulatory compliance of financial services has necessitated use of Data Quality and Data Governance strategies to re-invent the traditional financial services.
One of the SAS users group conducted a Case Study on National Bank of Canada, where the SAS system was used to design a credit-risk management system. National Bank of Canada’s Financial Group provides “financial services to retail, commercial, corporate and institutional clients.” They found the SAS System proactive, fast, and adaptable.
The Data Quality Dimension “Coverage” is the Most Prominent for AI Outcomes describes how “coverage” used as a DQ Dimension can prevent bad or wrong data to surface in ML use cases for the financial services sector.
Use Case for Data Governance: Risk Analysis
A widely used Data Governance application is risk management. Data breaches are common, and industry leaders are well aware of the adverse consequences of data breaches. The Top Five Data Governance Use Cases and Drivers describes how IT departments are proactively managing their “data-related risks” by adopting Data Governance 2.0 approach. According to the Trends in Data Stewardship and Data Governance Report, almost 98 percent of organizations have accepted the importance of Data Governance in assessing and managing data-driven risks.
Data Governance for Avoiding Swamps in Data Lakes
The data lake has become a data-storage repository of choice as it can hold very high volumes of multi-format (structured, semi-structured, and unstructured) data. Data Governance allows the data to be “tagged,” which helps users uncover contexts very easily while searching for relevant data for a specific purpose. This tagging mechanism also helps verify the quality, view a sample, and get a historical account of past actions on the data. To avoid a swamp, the data also needs to be strictly governed in terms ownership, accountability, sharing, and usage.
Data Quality and Data Governance for AI Outcomes
In most AI systems, the efficiency and impact of the predictive models depend on the scale and diversity of the data as well as on cleanliness of the data. Even the most powerful AI system may fail to deliver the expected results if the used “data” is not adequately governed and passed through quality checks. Thus, all AI-enabled business analytics systems must also be exposed to sound Data Quality and Data Governance frameworks to operate at maximum efficiency levels.
Data Quality & Data Governance can Maximize Your AI Outcomes describes how Data Quality and Data Governance can enhance the “predictive efficiency” of ML algorithms.
Data Quality Use Cases
A Talend blog post describes the use of Talend Data Quality solutions in six different industry verticals, which assure that in coming years, DQ platforms and tools will penetrate the global markets in a big way.
Common Applications of Data Quality Tools
Data Quality Study Guide — A Review of Use Cases & Trends states that
“It appears there is an abundance of data, but a scarcity of trust, and the need for data literacy.”
According to figures available from a Gartner, “C-Level executives believe that 33% of their data is inaccurate.” To gain the trust of both the employees and customers, these enterprises must turn to Data Quality tools.
A Syncsort blog post describes very common situations where Data Quality checks are performed without most people being aware of it
- Erroneous Addresses in Databases: In many cases, hardcopy forms may be used to collect the address data, which leads to handwritten and erroneous data. Sometimes, even online forms have many mistakes, generating a collection of low-quality data.
- Incomplete Phone Numbers: Phone numbers received directly from consumers are very often provided in haste and usually incomplete. This happens when the information provider does not know which components of the number (country code, area code, etc.) they are supposed to provide.
- Missing Field Entries: This happens very often while filling online forms. Users either miss certain field or enter field data in an incorrect format. Form designers have to take particular care to ensure that the fields provide information on entry format and that empty fields are flagged during form submission.
In the above cases, Data Quality tools are used to catch and rectify errors. Your Data Quality Situation is Unique (But it Really isn’t) talks about data profiling, which is how businesses choose to “organize, maintain, and utilize” their data. Data profiling makes every business’s DQ situation unique,and business users need to be aware of it. Data Quality Use Cases describes the SAS data-cleanup solution used in at least five different situations.
Conclusion
As global businesses continue to rely on data-driven solutions and AI systems for enhancing their competitiveness, Data Quality and Data Governance platforms will assume increased importance in the business landscape. The scale and volume of data-management solutions with a focus on quality and governance flooding the markets in the next few years may be surprising.
Image used under license from Shutterstock.com