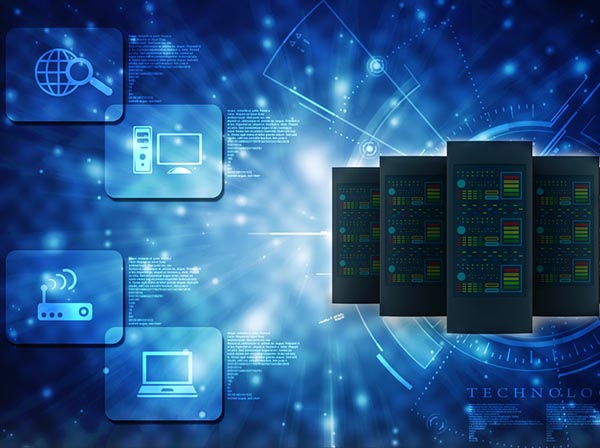
The level of sophistication around data has increased quite a bit in the 20 years Ron Agresta has been working with SAS. “Back in the day, it would be no governance, minimal quality, and those capabilities were not well managed. Now the whole segment has shifted,” he said, and it’s much rarer to find companies of any size that don’t grasp the importance of data. “The level of awareness about data within companies is also on a spectrum,” he remarked in a recent DATAVERSITY® interview. There are digital natives working with people who are very limited in their data use and understanding.
He discussed a customer that’s been in business for a long time and the company has processes that run calculations for insurance rates, perform billing operations, and other basic functions. The same company has another part of their business that is much more into aggregating data, doing analytics, trying to figure out what businesses they should be in, and how they can make more revenue. “So we’re seeing both the spectrum outside, across companies, and also within companies.”
Current Challenges
The biggest hurdle that some of Agresta’s customers are facing is in the area of data integration. Because SAS has been doing data integration for such a long time, they assumed at first that the integration problem was solved. “Well, that is true, but not with things like moving data to the cloud,” because he now has customers moving some—or all—of their data and related data processes to the cloud. “We have these hybrid environments where it’s a little bit more challenging to do traditional data integration processes,” he said.
And when Data Governance starts to come into play, it brings a new level of complexity to the integration piece. “It’s almost like ‘what’s old is new again.’ It’s still data integration, but the flavor of it has changed,” because there are more types of data coming from more different sources of data. Data resides in multiple different locations, “And so we’ve got to go refight and re-win that battle on the integration side,” he said.
Pain Points
Agresta said that Data Quality is a major source of frustration for his clients. In 2019 AI Predictions from Forrester,” 61 percent of survey respondents said that Data Quality was the biggest barrier to successfully implementing an AI project, and Agresta agrees. “It’s no big surprise that if you don’t have the basics like Data Quality under control, then whatever magic you’re expecting on the analytic side is not going to be what you anticipated.”
Once the data gets blended correctly, it is standardized, accurate, and up-to-date, and it’s really possible to see the results, he said. Moving beyond the hype of machine learning and AI, he sees clients deciding if and where technologies like natural language processing fit into broader enterprise Data Management and analytics projects that will extract the most value. It’s important for organizations to get the correct balance of “offensive” (being agile and exploratory with data) and “defensive” (Data Governance and control of data) approaches to solving data-centric problems.
Introspective Analytics
More companies are looking at automation and Agresta sees a need to scale Data Management processes without adding substantially more staff. Simply adding more people won’t allow organizations keep up with the continued flow of data. Using advanced analytics paired with automation can ease the burden on overworked data engineers and data stewards. Analytics can now suggest actions that can be taken on data to improve it without someone having to manually analyze loads and loads of data.
As an increasing number of users adopt the suggestions that are being made to them, the system starts to learn from those actions, and it can start to automate. “And when it sees something familiar, like a data set that looks like the data set you spent hours last week transforming, then it knows the kinds of things that it can do to improve that data.”
This ability to use analytics for improvements in internal processes can play an important part in self-service enablement, or self-service data preparation, where users may not have a robust skill set. “They have the data, they want to use the data, but they don’t have the technical expertise to do a lot of sophisticated things. But if the system can help them, then it gets them to doing the real work much quicker,” he said, which empowers users to do things like report building and advanced analytics.
This introspective process isn’t limited to Data Management, but can be used for other areas, such as Data Governance or quality improvement. Using analytics this way isn’t new, but Agresta said it’s starting to snowball as customers are learning about what is possible. And although SAS isn’t the only vendor in this area, he believes they have the advantage of decades in Data Management analytics. Their solutions portfolio arises from a desire to use the best from an advanced analytics perspective—whether that’s artificial intelligence, machine learning, advanced scoring, “Or whatever it might be—and to automate those solutions, making them less onerous to run and use.”
Data Protection
Extra scrutiny on data collection and usage has put many businesses on defense. Many companies rely almost exclusively on monetizing data relinquished by users, but regulatory attention is increasing in this area. “Seventy-three percent of consumers in the U.S. are very interested in knowing what companies are doing with their data and being able have some control over what companies are doing with that data.”
As organizations are coming to understand how important that is to their customers, it is becoming a major concern for companies:
“If we are doing an analytic process to audit what the inputs are, what the output is, how old the model is, who worked on it, and any other influential factors, it’s no longer unusual for regulations to require an explanation of those answers. It doesn’t have to be down to the if/then/else sort of thing, but it needs to be transparent enough.”
In 2019 and beyond, expect more laws for consumer data protection with the associated changes to technology needed to cope not far behind, he said. He also predicts an increased desire for transparency regarding how data is being collected, aggregated, and shared. This will call for enhanced technology that can deliver detailed reports to organizations and their customers about data usage.
Meaningful Results from New Technologies
Agresta predicts that more organizations will attempt to use AI and machine learning techniques to improve Data Quality and Data Management processes, but they will struggle to see meaningful results. Some companies wanting to adopt AI and machine learning are taking the “throw something at it and see if it works” approach rather than a more deliberate approach to solving key problems. “Anything that we can do to help our end-users make the right decisions about what algorithms to use and how to interpret the results is important.”
It can be easy to inadvertently combine data that shouldn’t be combined before even getting to the analytics, he said, and it’s essential that the company has the right level of data analytic capability before a decision is made on how to move forward with a report, or some other analytic-driven result.
“It’s easy to pick up any old analytic model, or process, or algorithm and apply it incorrectly, so we can’t belittle the fact that our tools are sophisticated, and we have to help our end-users use them in the right way.”
New technologies should be built on a solid foundation in order to get meaningful results. Understanding and using correct and approved statistical models to deal with outliers, for example, will directly impact the outcome. “In the simplest case, if you have outliers that you’re not dealing with in an appropriate way, whatever comes out at the other end is not legitimate.”
The Challenge of Data Governance
Data Governance is a growing challenge as more data moves from on-premise to cloud locations and governmental and industry regulations, particularly regarding the use of personal data. hybrid cloud, or hybrid Data Management systems must be able to communicate with each other about where data resides, what it contains, and who can access it.
SAS, historically, has developed solutions for metadata integration issues, and they are now also involved with an open source project called Egeria which is part of an ODPi initiative working to solve that problem.
“We’re working with other technology companies like IBM to come up with an open and bidirectional way to share metadata across independent technologies. I think that will go a long way.”
He sees Egeria as a great way to start solving some of the problems companies face about what data they have, how it works with other data they have, who is allowed to see it, where it came from, how old it is, and any number of other attributes that might be associated with that data.
Volume of Data vs. Data Sources
The challenge of data volume seems to be less troublesome for the majority of Agresta’s customers. He says that only around 10 percent are struggling with volume, and those could easily be handled by modest investments in technology. The remaining 90 percent are looking at the types of data becoming more of a challenge.
“If you think of structured databases, we’ve got that covered, and that’s a known, well-worn track. When you start to get to semi-structured, unstructured data, data streams, all kinds of different data sources, how do those worlds combine?” So it’s not necessarily data volumes that pose the biggest challenges, but what’s hidden in the data (good or bad) that can be difficult to deal with.
Agresta predicts we will continue to see an increasing use of more advanced analytics capabilities to solve complex problems that in years past might have taken large teams and years of research. Advanced analytics paired with good Data Management technology can help detect threats and uncover untapped opportunities.
What Agresta sees as a priority for SAS going forward is about making the end-user’s life easier, whether that means helping with the safe integration of cloud or hybrid stores, ensuring AI and other technologies are able to use quality data, or by helping their customers take advantage of advanced analytics: “Pushing the boundaries in the places that make sense. Seeing what’s successful, but not losing sight of the impact across the organization.”
Image used under license from Shutterstock.com