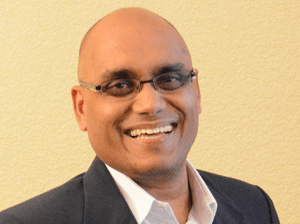
We’ve all heard of the many horrors of poor data quality. Companies with millions of records with “(000)000-0000” as customer contact numbers, “99/99/99” as the date of purchase, 12 different gender values, shipping addresses with no state information, etc. The cost of “dirty data” to enterprises and organizations is real. For example, the U.S. Postal Service estimated that it spent $1.5 billion in processing undeliverable mail in 2013 because of bad data. The sources of poor data quality can be many but can be broadly categorized into data entry, data processing, data integration, data conversion, and stale data (over time).
So what can you do to make sure that your data is consistently of high quality? There is increasing awareness of the criticality of data to making informed decisions and how inaccurate data can lead to disastrous consequences. The challenge lies in ensuring that enterprises collect/source relevant data for their business, manage/govern that data in a meaningful and sustainable way to ensure quality golden records for key Master Data, and analyze the high-quality data to accomplish stated business objectives. Here is the six-step Data Quality Framework we use based on the best practices from data quality experts and practitioners.
Step 1 – Definition
Define the business goals for Data Quality improvement, data owners/stakeholders, impacted business processes, and data rules.
- Examples for customer data:
- Goal: Ensure all customer records are unique, accurate information (ex: address, phone numbers etc.), consistent data across multiple systems, etc.
- Data owner: Sales Vice President
- Stakeholders: Finance, Marketing, and Production
- Impacted business processes: Order entry, Invoicing, Fulfillment etc.
- Data Rules: Rule 1 – Customer name and Address together should be unique; Rule 2: All addresses should be verified against an approved address reference database etc.
Step 2 – Assessment
Assess the existing data against rules specified in Definition Step. Assess data against multiple dimensions such as accuracy of key attributes, completeness of all required attributes, consistency of attributes across multiple data sets, timeliness of data, etc. Depending on the volume and variety of data and the scope of Data Quality project in each enterprise, we might perform qualitative and/or quantitative assessment using some profiling tools. This is the stage to assess existing policies (data access, data security, adherence to specific industry standards/guidelines, etc.) as well.
- Examples:
Assess % of customer records that are unique (with name and address together); % of non-null values in key attributes etc.
Step 3 – Analysis
Analyze the assessment results on multiple fronts. One area to analyze is the gap between DQ business goals and current data. Another area to analyze is the root causes for inferior data quality (if that is the case).
- Examples:
If customer addresses are inaccurate by more than the business-defined goal, what is the root cause? Is the order entry application data validations the problem? Or the reference address data inaccurate?
If the customer names are inconsistent between order entry system and financial system, what is causing this inconsistency?
Step 4 – Improvement
Design and develop improvement plans based on prior analysis. The plans should comprehend timeframes, resources, and costs involved.
- Examples:
All applications modifying addresses must validate against selected address reference database; Customer name can only be modified via order entry application; The intended changes to systems will take 6 months to implement and requires XYZ resources and $$$.
Step 5 – Implementation
Implement solutions determined in the Improve stage. Comprehend both technical as well as any business process-related changes. Implement a comprehensive ‘Change Management’ plan to ensure that all stakeholders are appropriately trained.
Step 6 – Control
Verify at periodic intervals that the data is consistent with the business goals and the data rules specified in the Definition Step. Communicate the Data Quality metrics and current status to all stakeholders on a regular basis to ensure that Data Quality discipline is maintained on an ongoing basis across the organization.
Data Quality is not a one-time project but a continuous process and requires the entire organization to be data-driven and data-focused. With appropriate focus from the top, Data Quality Management can reap rich dividends to organizations.