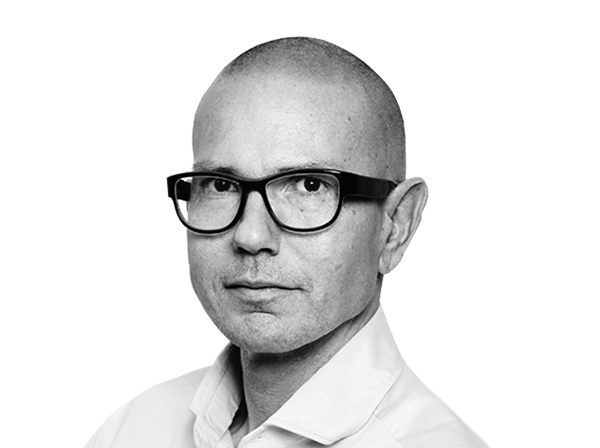
The abilities of an organization towards capturing, storing, and analyzing data; searching, sharing, transferring, visualizing, querying, and updating data; and meeting compliance and regulations are mandatory for any sustainable organization.
Many companies have already invested in their data environment by deploying a traditional data warehouse, but data warehouses have many limitations.
For example, most data warehouses are typically updated as an end-of-day batch job, rather than being filled by real-time transactional data. And in a structured data warehouse environment, work must be done within the framework of the created structure: static data sets with minimal ability to drill down. Data warehouses tend to take an exorbitant amount of time to build and are very costly. Furthermore, many organizations have been disappointed that once their data warehouse finally goes live, it does not meet their intended business needs that they had hoped for when mapping out earlier objectives.
The requirement for something less costly and more efficient has led many businesses to move to a “modern data estate.”
So, what exactly is a “data estate”?
To help explain, I refer back to a definition I wrote for a previous Forbes article that states a date estate is simply: “The infrastructure to help companies systematically manage all of their corporate data. A data estate can be developed on-premises, in the cloud or a combination of both (hybrid). From here, organizations can store, manage and leverage their analytics data, business applications, social data, customer relationship systems, functional business and departmental data, internet of things (IoT) and more.”
The transition to a data estate is rather easy, especially when taking advantage of data automation, no code/low code, and best practices. Here are seven factors to consider for building and deploying a modern data estate.
1. Assessment of Current Analytics-Data Environment
Some organizations are still conducting analysis by using spreadsheets, while others have departments that have developed their own analytics systems; in either scenario, the reporting and analysis is below standards, as the company as a whole is neither data-holistic nor congruent. This means missing out on enterprise-analytics data or corporate programs such as AI and ML. It could also mean that users are taking action outside of corporate governance. Collecting and centralizing all this data into a data estate allows you to collect and centralize analytics data into a single, unified Data Management platform.
2. Define the Business Needs of Today and Tomorrow
Not having an enterprise data infrastructure that seeks to establish various metrics such as speed-to-data, agility to add in new data elements, and flexibility to address future needs can limit an organization’s ability to compete. With a data estate, you empower your organization to provide instant access to data and “one version of the truth” for reports as historical data is centralized.
When transforming into a modern data estate, a big mistake is merely replicating the existing environment into the new environment. It’s not about IT. It’s about focusing on business needs.
A modern data estate allows organizations to easily designate user access (see point #4) from the outset so that job tickets into IT are no longer needed. This enables users to have access to trustworthy data as needed, when needed, rather than waiting for IT approval.
3. Describe the Core Business and Data Processes
Identify the data sources you have available – and which data you want to initially find a home for in your data estate – and begin by responding to the highest priorities. This action is about business processes and data processes since both are connected. You’ll look at a certain data point, such as customer data, and then you’ll define the relational data models and determine how the customer data is used in your business processes. The same applies to transactions, products, and more.
4. How Will the Data Be Accessed and by Whom?
Employees are different in the data they have access to (security and control) and in the way they access the data (tooling). Describe your security strategy and the tools for analyzing, reporting, and visualizing data.
Some questions to consider:
- How are you going to connect to the data sources?
- What connectors do you need?
- How often can you read data?
- What kind of data do you get?
- What metadata is available?
- How often is data updated and how often do you have access to data?
- How will you manage security and access rights?
Consider the data consumers you want to serve and how you want to serve them. You want to provide self-service BI for different types of consumers, ranging from power users such as data scientists and data miners who rely heavily on AI and ML algorithms, to business users working ad-hoc with data and creating new reports, to casual users waiting for the routine reports and updated dashboards.
You’ll define the roles and groups so that when building your data estate, you can identify appropriate users for appropriate access rights. This ensures that authenticated users only access the data, tables, or columns they are authorized to see.
5. Define Your Architecture
Data needs to be extracted, processed, and refined to be useful. And just as oil can be refined into different types of fuel, data can be prepared for different uses when it comes to analytics and AI.
Outline how your organization chooses to prepare data for these different uses, from reporting to analytics to artificial intelligence. Most data estates are split into three distinct layers: the data lake, the data warehouse, and data marts.
- Data Lake: This layer is primarily for power users such as data scientists, who perform various types of analysis on raw data to look for anomalies and patterns, and eventually perform machine learning. This layer enables quick ingestion of raw data from all data sources and into Azure Data Lake or a SQL Database.
- Data Warehouse: Raw data isn’t the best choice for business users, such as business analysts. These users need data that has been cleansed, enriched, and rationalized – in a modern data warehouse. In a layered Data Architecture, this data warehouse would be sourced from the data lake – but placed in a SQL-based database with semi-structured data transformed into structured data for analysis.
- Data Mart: The data mart supports common users by delivering relevant datasets from the data warehouse, enabling self-service analytics across multiple analytics tools for line of business or function-specific views, so that business users can explore data safely and efficiently.
6. Cloud, On-Premises, or Hybrid?
You don’t want your data estate to end up becoming a costly affair to maintain. Some think to manage costs they should simply “go to the cloud.” Instead, evaluate the pros and cons of cloud, on-premises, or hybrid. Think long and hard about defining a clear purpose and specific needs for going to the cloud rather than simply deploying.
7. Select Your Construction Partners
Consider who will build the data estate. Which software will you use for your data estate? How will you maintain the estate?
Data Management and Automation Software: You’ll want to select the right software platform for today and tomorrow to ensure that your data estate is built with an integrated Data Management platform that is completely independent from developers, data sources, data platforms, front-end tooling, and deployment model.
You should be able to expedite development with automated code-generation, freeing data engineers to focus on Data Quality and business requirements to limit the required number and types of highly skilled resources by using a single tool to build your data lake, data warehouse, and data marts.
You’ll also want to ensure you data estate is “future-proof,” meaning it is fully scalable and ready to adopt future releases without rebuilding.
Deployment and Maintenance Partner: Will you deploy and maintain the data estate yourself? Will you consider a deployment partner and then take on the maintenance yourself? Many organizations opt for this as they want to ultimately take control of their data and put it in their own hands, without having to depend on a business partner. Whatever you decide, consider a partner with experience and who you can trust, as they will be deploying a future-proof foundation for your most valuable asset: your data.
Closing Thoughts: Think Big, Start Small, and Act Agile
If you have accounted for these seven factors, you’re well on your way. It’s very likely that your data estate will help drive innovation and that you will be deploying a scalable, future-proof data environment.
One final note: It’s key to start small. For example, when developing an estate in the cloud (which you can scale to production), do so with only a couple of data sources, a few tools, and then test and experiment.
If you go one step at a time, you’ll help your company transform into a data-driven organization, while benefitting from greater efficiency and lower costs from your data program. You’ll also recognize that your company is making faster, better business decisions to help you become more successful.