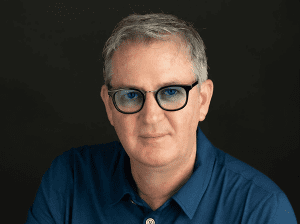
Enterprises all around the world are relying on analytics to provide the insights they need to streamline operations and delight their customers. However, making the right data available to the right people at the right time is becoming more and more challenging. While the ability to perform analytics on huge volumes of data is beefing up business performance, it also comes with certain risks that need to be avoided. Here are some of the latest data democratization trends that enterprises are experiencing while they struggle to provide fast and safe access.
ChatGPT Data Breaches: According to cyber-intelligence firm Group-IB, more than 101,000 ChatGPT user accounts were stolen by malware over the past year. Open AI, the creators of ChatGPT, reported that due to a software bug, approximately 1.2% of the ChatGPT Plus subscribers who were active on March 20, 2023, between 1:00 a.m. and 10:00 a.m., had their data exposed, which adds up to approximately 1.2 million users. In addition to these two incidents, ChatGPT is generally susceptible to breaches because data is entered through a web browser enabling sensitive information that is stored on the network to be exposed to hackers. Also, data entered into a ChatGPT prompt used to train the next version of the algorithm can be hacked by an intruder if the right security precautions aren’t put in place.
Focus on Data Discovery: While previously companies had central data repositories, today they have dozens of data stores, pipelines, and private and off-premises clouds. It’s becoming increasingly difficult for enterprises to identify which data they have and where it’s located. The data discovery process combines these individual data sources to create a central virtual data repository that can be accessed centrally to manage data access. By performing automatic polling on a regular basis, data discovery can reveal hidden sensitive data stored in systems not managed or authorized by IT. Today, due to shadow IT, many organizations lack a single source of truth for their data making analytics and reporting inaccurate, inconsistent, or incomplete while degrading the quality of insights produced from this data and introducing compliance issues.
Automatic Policy Generation: Businesses all over the globe are grappling with the complex and highly dynamic regulatory landscape. Over 130 countries have put in place legislation to secure the protection of private data. In addition, specific business units within a business organization may be subject to their own industry-specific compliance standards. Businesses are urged to review and update their privacy policy at least once every 12 months, or even more frequently if the business and its data practices are dynamic – no matter how small the revisions are. The process of tracking, analyzing, and then incorporating regulatory changes to generate a data access policy, is becoming automated to reduce the man-hour requirements and the risk of human error.
Centralized Data Access Management: As enterprises reach a higher level of data maturity, having centralized Data Governance is becoming the best way to enforce compliance and internal security policies. Data silos make it impossible to gain a clear unified view of business data. In addition, organizations that house data in multiple systems face challenges maintaining data security. Centralizing data access creates a less complex environment for data, making it easier to monitor and control the use and protection of data. Centralized access control consolidates access control management into a central system that determines permissions based on predefined policies, and international, local, and industry-specific compliance standards and enables access events to be logged and monitored in real-time to confirm compliance with regulations.
Self-Service Data Analytics: Self-service analytics platforms enable non-technical users to access, analyze, and visualize data without requiring assistance from data experts or IT teams. Providing self-service analytics improves accuracy, agility, and efficiency for decision-making by delegating analytics tasks directly to the business users who are experts in their field or sector, and who have a deeper understanding of their data. This trend is continuing to grow, making data available to more employees and allowing business users to make data-driven decisions independently minimizing their reliance on opinions or gut feelings. In addition to providing the necessary tools there is a movement within enterprises to educate users about the value of data and how to create and use the analytics, they need to make more informed business decisions.
Data Mesh Culture Transformation: At the same time that security and compliance governance is being managed centrally, there is a movement to enable separate regions and business units to execute policies locally. Data ownership, responsibility, and governance are often distributed across different domains or teams within an organization. For example, titles for employees who have responsibility for data security can include data engineers, analysts, analytics engineers, data scientists, product managers, business analysts, and citizen data scientists from different business units. In order to provide consistent protection and flexibility there is a current trend to implement platforms that can be utilized at different endpoints while enforcing data access policies that are defined at a centralized location.
Increased Adoption of AI in Data Governance: The integration of AI and machine learning technologies into Data Management has become a groundbreaking advancement in how an organizations handle their data and govern their data-related processes. AI is being used to identify inconsistencies duplicates and errors within large datasets and to reduce the manual effort required to clean data. In addition, data access patterns are being analyzed to proactively recommend new angles of investigation to respond to a specific query, and to automatically scale analytics up based on predicated data usage.
Analyzing data to unveil market trends and customer preferences has become an essential practice for businesses to keep a competitive edge. Due to the intricate data ecosystem and the ever-evolving regulatory landscape, organizations must establish robust data governance. The surge in data volumes, and the emergence of new technologies have introduced a level of complexity that demands more advanced methods of data access management. Intelligent automation can match the pace of change ensuring the decision-makers obtain the best possible business intelligence while ensuring a company’s regulatory compliance.