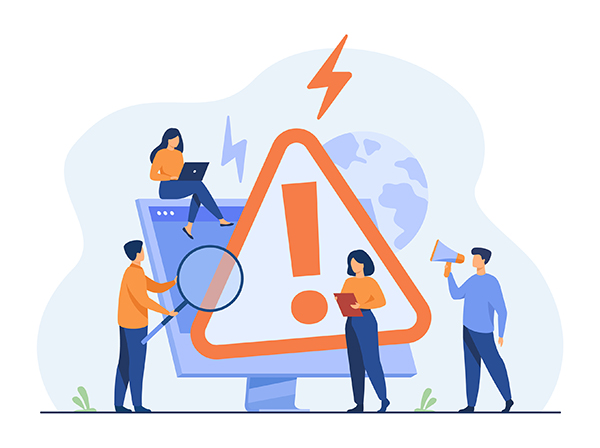
Having Data Quality issues is a common – and costly – problem. According to Gartner, poor-quality data costs organizations an average of $12.9 million annually. Data Quality uses factors such as accuracy, consistency, and completeness in determining the value of the data. High-quality data can be trusted, while low-quality data is inaccurate, inconsistent, or incomplete. In addition to significant amounts of lost revenue, using low-quality data can result in poor business decisions and reduced operational efficiency.
Poor-quality data will weaken and damage important business activities, such as running email campaigns and identifying repeat customers.
Clean, accurate, high-quality data allows an organization to make intelligent decisions and accomplish goals. The better quality the data, the more probable it is that sales and marketing efforts will be successful. The impact of poor Data Quality on sales and marketing can include such things as unreliable customer targeting or unpleasant customer experiences.
Additionally, poor Data Quality can prevent automation from working properly.
There are a variety of ways sales and marketing advertising can be automated. But, because automated advertising campaigns rely on high Data Quality (or accuracy), they can alienate potential customers if that data is instead poor quality.
Unfortunately, fixing Data Quality issues isn’t a once-and-done activity. It is a process requiring continuous attention.
Data Governance: Responsibility and Technology
Generally speaking, Data Governance programs, which are a combination of technology and human behavior, are responsible for Data Quality – as well as complying with various regulations. Software is commonly used to provide automated services for processing the data, while humans need to be trained in the best ways to promote high-quality data.
Having a single person, the data steward, be responsible for the education of staff and the maintenance of the program overall is an efficient way of promoting high-quality data.
The data steward is responsible for educating the staff on how to support good Data Governance, and assuring the software is working appropriately. (In many organizations, the data steward reports to the chief data officer, who in turn reports to the Data Governance committee.)
A well-designed Data Governance program, which includes human intervention, will correct poor Data Quality issues.
Common Data Quality Issues, and How to Deal with Them
Poor Data Quality promotes bad decision-making. Having high-quality data promotes good decision-making. It is important to resolve Data Quality problems as quickly as possible. Some Data Quality issues are more common than others, and are listed below:
Data inconsistencies: This problem occurs when multiple systems are storing information without using an agreed upon, standardized method of recording and storing information. Inconsistency is sometimes compounded by data redundancy. For example, a customer’s last name being recorded before their first name in one department, and vice versa in different departments. Yet another problem is when one department stores data in a PDF format, while another uses Microsoft Docs.
Fixing this problem requires the data be homogenized (or standardized) before or as it comes in from various sources, possibly through the use of an ETL data pipeline.
Incomplete data: This is generally considered the most common issue impacting Data Quality. Key data columns will be missing information, often causing analytics problems downstream.
A good method for solving this is to install a reconciliation framework control. This control would send out alerts (theoretically to the data steward) when data is missing.
Orphaned data: This is a form of incomplete data. It occurs when some data is stored in one system, but not the other. If a customer’s name can be listed in table A, but their account is not listed in table B, this would be an “orphan customer.” And if an account is listed in table B, but is missing an associated customer, this would be an “orphan account.”
An automated service that checks for consistency when data is downloaded into tables A and B is a potential solution. Finding the source of the problem (often a human) is another option.
Irrelevant data: Irrelevant data is everywhere. Screening it out in advance, before storage, can be time-consuming, and may eliminate data that “could be” useful. Unfortunately, storing great chunks of data is more expensive and less sustainable than making the effort to screen out the useless data in advance. Screening out the useless data is more efficient and cost-effective from a big-picture perspective.
To solve this problem, setting limits (sometimes known as data capturing principles) should become a research requirement. Broadly speaking, if the data can be used to accomplish an end goal, it’s fair game. If not, the data should not be collected.
Old data: Old data, like old information, loses value, and over time will no longer represent reality. Things change. Storing old data is an unnecessary expense. It can confuse staff, and it has a negative impact on performing data analytics. Storing data after a certain amount of time offers no value and promotes data decay.
The Data Governance software should have a “GDPR principle on retention” option, which can be set to save it for “no longer than necessary.”
Redundant data: On occasion, multiple people within an organization will capture the same data, repeatedly. Not only is this a waste of staff time (six people collecting the same data, when only one is needed), but there is the expense of storing the redundant data.
A master data management program can be used to resolve this issue.
Duplicate data: When data is duplicated, it is stored in two or more locations. Normally, this isn’t much of an issue, unless the duplicated data is “old,” of poor quality, or being duplicated multiple times. While fairly easy to detect, it can be a little difficult to fix.
For relational (SQL) databases, there is a feature called “normalization” that can be used to deal with duplications. Additionally, master data management controls can be implemented to support a “uniqueness check.” This control checks for exact duplicates of stored data and purges one (or more) duplicates.
Best Practices for Data Quality
Using best practices can act as a form of preventative maintenance and help to avoid Data Quality problems.
- Automation: Cloud computing makes it easy to access data from several different sources, but also comes with the challenge of integrating data from different sources and in different formats. Dealing with this challenge requires the data be cleansed and de-duplicated. (Typically, a data preparation tool is used to reduce the amount of human labor.)
- The necessity of general consensus: If only 75% of a business’s staff are committed to ensuring good Data Quality, then it is reasonable to expect “some” of the data will be of low quality. All of management, and all the staff dealing with data, must understand the importance of Data Quality and take responsibility for maintaining it. This is where the data steward comes in – first, as an educator and, when needed, as the data police, to enforce Data Governance policies.
- Measuring Data Quality: A formula has been developed that allows for rough measurements of an organization’s Data Quality. By creating a measurement system to determine the quality of the data, and using it, problem areas can be identified and corrected, resulting in higher-quality data. This can be scheduled as a monthly Data Quality audit. Measuring Data Quality is not the same as correcting the errors. It simply clarifies which areas are having problems.
- Developing a Data Governance program: If the business does not already have a Data Governance program, it’s probably time to develop one. A Data Governance program can be described as a collection of policies, roles, processes, and standards that promote the efficient use of data for achieving the business’s goals.
- Educating staff and management: This should be organized by the data steward, with the help of the chief data officer. Since homework generally isn’t an option, time will have to be scheduled during work hours. This could be done for a few hours, with almost everyone attending, or it could be done with small groups of staff, or some combination of the two.
- A single source of truth (SSoT): This concept helps to assure all staff making decisions are using the same predetermined, highly trustworthy source. Many critical business decisions rely on accurate, high-quality data, and using a trusted source will minimize mistakes. An SSoT is typically one centralized storage area for all the business information. (Some research data will have to come from outside sources, but info about the business should come from the SSoT.)
Conclusion
Poor Data Quality can have a tremendous impact on important research projects, such as business intelligence and developing the customer experience. Fixing Data Quality problems should be one of the organization’s top priorities, and intelligent investing in it will improve efficiency and increase profits.
Image used under license from Shutterstock.com