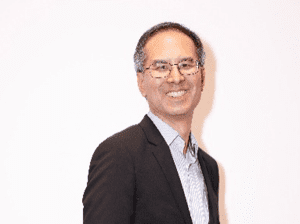
As data science professionals, we are often viewed as people who draw conclusions based only on data and minimize other factors. This perception usually becomes contentious when the insights and evidence from the data are inconsistent with somebody else’s “hypothesis.” Or we are confused and maybe frustrated when “qualitative” analysis trumps quantitative analysis. The next time you feel this frustration, consider these four perspectives on data analytics to validate and consider other views so you can try and find common ground:
1. “Outliers equal opportunity.”
Outliers present themselves in a dataset as anomalies. Maybe outliers are noise, but maybe they are special.
Outliers could be unique insights, emerging trends, or interesting segments. In medical research, an outlier could point to a rare but life-threatening side effect of a medication. In the case of customer data, an outlier can be a valuable customer niche that has not yet been addressed. Outliers could be an emerging trend. The color pink started off as an outlier but quickly became the most popular fashion choice.
Before dismissing outliers as noise, use them to spark questions and curiosity:
- Does the outlier point to an opportunity?
- Why does the outlier exist?
- If you could change the time stamp of your data set, how could that impact the outliers?
- Would you have to assume if there are more outliers?
- What does an outlier tell us about the system or process being analyzed?
- What would it take for an outlier to become a distinct profile or segment?
Understanding outliers can lead to innovative product development, identifying new market opportunities, and recognizing potential risks. In fields such as environmental science or economics, outliers can signal important pattern changes, like sudden climate shifts or financial crises. Outliers have the potential to transform the way we view and interpret data, changing them from misunderstood data points to valuable gems of information.
2. “Once is happenstance. Twice is a coincidence. Three times is enemy action.” –Goldfinger
Ever wonder why others are comfortable making “data-driven” decisions with very limited information? More data points give us all more confidence and higher accuracy, but sometimes, we need to act quickly.
Most recently, OpenAI launched ChatGPT despite its flaws, while others who had similar products waited to increase their confidence level in the accuracy of responses. When you think somebody is making a data-driven decision with low confidence levels and limited accuracy, consider the cost of time. The enemy may be firing.
3. “Not everything that counts can be counted, and not everything that can be counted counts.” –commonly attributed to Albert Einstein
In other words, “I appreciate your data analysis, but what I think or hear is more important. It can’t be counted or measured.”
How do you respond? This situation is where you need to get creative.
For example, customer behavior, including customer sentiment, brand loyalty, and trends driven by cultural shifts, can be intangible and difficult to quantify. If you only have online behavior data, use other methods to access new data sources such as test programs, surveys, social sentiment analysis, online ethnography, or back-to-the-basics primary customer research.
Maybe nothing will be definitive, but it is the combination and consistency of different methods and sources that point to a consistent conclusion.
4. “Correlation equals causation?”
Substituting correlation for causation can lead to misguided decision-making when done without awareness. However, there are situations where we only have access to correlation data. In these cases, it’s critical to scrutinize whether the correlation is mere coincidence or if there’s a valid underlying cause.
For instance, consider the challenge of measuring marketing spend attribution and analyzing sales activities. These are complex tasks with no direct causal link. One might observe a 90% closing rate when customers visit a vendor’s office for a customer briefing, but it’s important not to jump to conclusions and assume causation. Instead, a more nuanced approach is needed.
Upon closer examination, it becomes evident that the high closing rate is not a result of simply scheduling customer briefings for every sales interaction. Instead, the interactions themselves create the desire in clients to attend these briefings, which subsequently leads to a high closing rate. This example illustrates the fusion of art and science in analytics – a process that involves understanding the underlying dynamics and not just relying on superficial correlations.
We’d all like the statistical confidence of lots of data with the ideal dataset. The reality is that sometimes, we must get creative and imaginative and examine outliers, correlations, and alternative datasets. Or sometimes, there is no time, and you need to act on limited data.