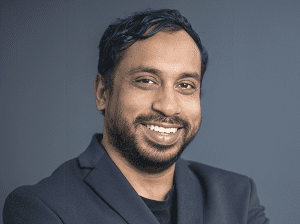
As the digital world grows increasingly data-centric, businesses are compelled to innovate continuously to keep up with the vast amounts of information flowing through their systems. To remain competitive, organizations must embrace cutting-edge technologies and trends that optimize how data is engineered, processed, and utilized. From decentralized frameworks to AI-driven advancements, 2025 is poised to be a transformative year for data engineering.
We delve into the nine critical trends shaping the future of data engineering and how businesses can leverage them for success.
1. Generative AI: Redefining Data Management
Generative AI is taking data management to the next level by automating tasks like data cataloging, governance, and anomaly detection. It’s also making data more accessible through natural language querying.
Advanced Capabilities
In 2025, generative AI tools will offer:
- Dynamic Schema Generation: Automatically creating and adapting data schemas based on new requirements.
- Natural Language Interfaces: Enabling users to interact with data using conversational queries.
2. Breaking Down Silos: The Data Mesh Revolution
The data mesh architecture is disrupting traditional centralized data management systems by decentralizing data ownership. Instead of relying on a single team to manage all data, data mesh distributes responsibility to domain-specific teams, treating data as a product. This approach fosters greater collaboration and ensures that teams have direct access to high-quality, domain-relevant data.
Why It Matters
Large organizations, with their sprawling departments and data requirements, often struggle with bottlenecks in centralized data management. By adopting data mesh, they can empower each domain to manage and maintain its own data, improving agility and scalability.
Real-World Applications
Enterprises in finance, healthcare, and manufacturing are early adopters. For example, a multinational bank using data mesh can enable its credit risk team to access and analyze real-time loan data without waiting for central IT to process requests.
Challenges and Solutions
While data mesh provides autonomy, it risks creating silos if not properly managed. Interoperability tools and standardized governance frameworks will be crucial in 2025 to ensure seamless data exchange across domains.
3. DataOps in Action: Automation Meets Agility
DataOps, an offshoot of DevOps, emphasizes collaboration, automation, and continuous improvement in data pipeline management. It addresses the inefficiencies and slow turnarounds in traditional data engineering processes.
The Rise of Intelligent Automation
In 2025, we anticipate significant advancements in AI-integrated DataOps tools. These will include self-healing pipelines that automatically resolve errors and predictive analytics to foresee data bottlenecks. For instance, an e-commerce company using AI-driven DataOps could detect and fix discrepancies in sales data in real-time, ensuring accurate reporting during high-traffic sales events.
Benefits Beyond Speed
- Reliability: Automated testing ensures pipeline stability.
- Collaboration: Teams can work simultaneously on data projects without conflict.
- Scalability: Businesses can easily scale operations without overhauling pipelines.
Organizations adopting DataOps will likely see improved efficiency, reduced downtime, and faster delivery of actionable insights.
4. From Compliance to Confidence: Reinventing Data Security
With data privacy regulations like GDPR, CCPA, and HIPAA evolving, organizations face mounting pressure to enhance their security protocols. A single breach can cost millions and erode customer trust, making data security and compliance top priorities.
Emerging Technologies in Data Security
- Tokenization and Masking: Protect sensitive data during processing without exposing raw information.
- Real-Time Compliance Monitoring: AI tools now offer real-time alerts for non-compliance, allowing businesses to address issues proactively.
- Privacy-Enhancing Computation: Techniques like homomorphic encryption and differential privacy will see increased adoption, enabling secure computations on encrypted data.
Practical Example
Healthcare providers are leveraging privacy-enhancing computation to analyze patient data for research without exposing identifiable information, ensuring compliance with strict regulations.
Forecast for 2025
Expect a stronger emphasis on adaptive security models that can dynamically adjust to threats and regulatory changes. Businesses that proactively adopt these measures will gain a competitive advantage in maintaining customer trust.
5. Smarter Pipelines: AI/ML Take the Driver’s Seat
AI and machine learning are transforming data engineering by automating repetitive tasks, such as data cleansing, schema mapping, and anomaly detection. These tools enhance the speed and quality of data processing.
Key Innovations
- Real-Time Data Enrichment: AI tools automatically add context to raw data, making it more useful for decision-making.
- Metadata Management: AI-powered solutions simplify the management of metadata, ensuring it remains accurate and up to date.
Transformative Impact
A retail company using AI for customer segmentation could dynamically adjust its marketing strategies based on real-time sales data, driving higher engagement and revenue.
What’s Next
In 2025, AI-powered pipelines will become smarter, with capabilities like predictive maintenance, where potential failures are detected and resolved before they occur. This level of foresight will be critical for industries like manufacturing and logistics.
6. Lightning-Fast Insights: Real-Time Analytics on the Rise
The ability to process and act on data in real time is becoming a competitive necessity. Businesses are leveraging streaming analytics tools to gain immediate insights.
Use Cases Driving Adoption
- Personalized Customer Experiences: Retailers use real-time data to tailor offers and recommendations.
- Operational Efficiency: Logistics companies track shipments in real-time to optimize delivery routes.
- Fraud Detection: Banks monitor transactions continuously to identify and block suspicious activities instantly.
The Role of Edge Computing
In 2025, edge computing will complement real-time analytics by processing data closer to its source, reducing latency. This is particularly relevant for IoT applications, where split-second decisions can have significant implications.
7. Beyond Visibility: The Era of Data Observability
Data observability goes beyond traditional monitoring, offering a comprehensive view of pipeline health and performance. This proactive approach ensures data reliability and pipeline stability.
Components of Data Observability
- Pipeline Monitoring: Tracks data flow and identifies bottlenecks.
- Data Quality Checks: Flags inconsistencies or anomalies in real-time.
- Root Cause Analysis: Identifies the underlying causes of issues, minimizing downtime.
2025 and Beyond
As data ecosystems grow more complex, AI-driven observability tools will emerge, offering predictive analytics to anticipate and prevent issues. For example, a telecommunications company could use these tools to ensure uninterrupted service during high-demand events.
- Building Without Boundaries: Low-Code/No-Code Empowerment: Low-code and no-code platforms are revolutionizing data engineering, enabling users to build data pipelines without extensive coding expertise. This democratization of data empowers business users to solve their own data challenges.
- Evolving Platforms: Next-generation low-code platforms will incorporate AI to assist users in designing more complex pipelines with minimal input. Integration with enterprise systems like ERP and CRM will also become seamless.
- Real-World Impact: A marketing team, for instance, could use a no-code tool to build a pipeline that aggregates customer data from multiple sources, enabling faster campaign launches without relying on IT.
- Broader Accessibility: By reducing the technical barriers to entry, these platforms will enable businesses to innovate faster while cutting costs.
8. Green Data: Engineering with Sustainability in Mind
Sustainability is becoming a non-negotiable aspect of data engineering. Companies are optimizing their data workflows to minimize energy consumption and reduce environmental impact.
Steps Toward Sustainability
- Energy-Efficient Algorithms: Optimizing data processes to use less computing power.
- Green Data Centers: Leveraging renewable energy and advanced cooling systems.
- AI for Resource Optimization: Tools that balance workload distribution to conserve energy.
Why It’s Crucial
Beyond cost savings, sustainable practices enhance corporate reputation and align with global environmental goals. Industries like tech, manufacturing, and logistics are leading this shift.
The Future Outlook
Generative AI will continue to expand its role, driving efficiency and innovation in data management. Businesses adopting these tools will see faster, more accurate data processing and better governance.
Preparing for the Future
To capitalize on these trends, businesses should:
- Invest in Training: Upskill teams in tools like data mesh, AI/ML, and low-code platforms.
- Adopt Agile Frameworks: Implement methodologies like DataOps for greater flexibility.
- Commit to Security: Stay ahead of regulations with proactive security measures.
- Embrace Sustainability: Align data operations with environmental goals.
- Leverage Real-Time Insights: Prioritize tools for streaming analytics and observability.
Conclusion: Thriving in a Data-Driven World
The trends shaping data engineering in 2025 offer exciting opportunities for businesses to innovate and excel. By embracing these advancements, organizations can build resilient, efficient, and future-ready data ecosystems that drive success in an increasingly data-centric world.