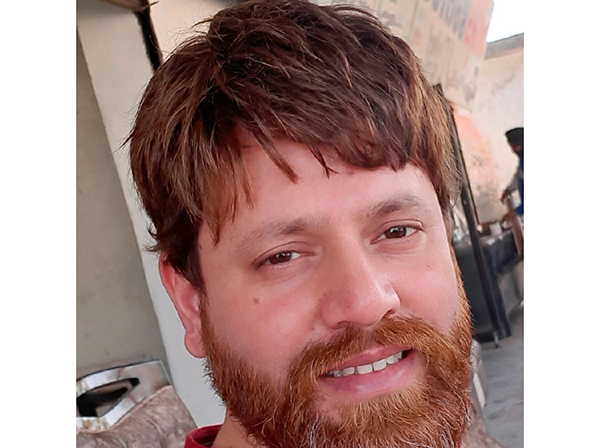
Data Science is a diverse field with an array of career and job options out there to pursue. The modern economy is dependent on data and data analysis so, naturally, data scientists are in high demand and enjoy good salary and job security prospects. With that in mind, below are 11 intriguing roles for data scientists in 2022.
Data Scientist
Data scientists do a lot of things, but in their purest form, they study data sets for insights that might help their company, using math and programming abilities. Typical daily duties include finding the right data sets and variables to investigate and solve a problem, collecting vast amounts of data from a variety of sources, finding answers to business challenges using data approaches, and working together with IT and business teams.
Data scientists also look for patterns and trends in data that might have an impact on the company’s strategy. A data scientist working for a manufacturing company, for example, might spend a lot of their time parsing data collected by facilities management software.
Data scientists can expect to earn highly competitive salaries right out of college, with career trajectories that take them well into the six figures. There will be a sustained need for people with strong computer and information research skills throughout this decade and beyond.
Machine Learning Engineer
Machine learning experts develop and run automated software algorithms that can produce prediction models from big data sets. The systems “learn” from the data gathered, allowing them to create more accurate prediction models. If you are curious about the basics of AI and machine learning, and some of their many uses, there are plenty of resources online to help you begin to build your knowledge base.
Machine learning engineers frequently create applications such as image and speech recognition software for activities like auto-tagging photographs and translating text to spoken dialogues. They help build customer insight programs that learn about customers’ activities and provide suggestions based on their preferences on a constant basis and work on algorithms capable of detecting fraud in real-time or evaluating massive amounts of historical data to generate financial forecasts that reduce risk for businesses.
Machine learning is one of the most exciting areas of AI and business automation and is heavily reliant on good data scientists.
Artificial Intelligence Engineer
Traditional machine learning approaches are used by artificial intelligence developers to construct models that fuel AI applications. AI engineers operate at a deeper process level, whereas data scientists look at things from a higher-level business viewpoint.
AI developers frequently rely on a wide range of skills, including programming, with python and R skills a major asset. They also need a solid understanding of arithmetic fundamentals in order to assess the accuracy of models, especially a strong understanding of statistics.
These data scientists can easily earn six figures, with job postings across industries around the world. AI is the future, and data scientists are responsible for much of AI’s current and future success.
Machine Learning Scientist
Machine learning researchers focus on developing algorithms that are employed in data models. They also work on the software engineering that will be utilized to accomplish them.
To land a job as a machine learning specialist you need the ability to do deep and exhaustive research, an ability to distinguish between dispersed signals and C or C++ coding skills, as well as other Data Science programming languages.
You also need a strong understanding of artificial intelligence, know how to create and execute automation, and be able to use data models. If you can do all of that, you can expect to make well over 100K a year. People who can build and augment the algorithms used in predictive data models are extremely valuable in the modern economy.
Data Architect
The vision that transforms fundamental business requirements into technology solutions comes, in large part, from data architects. They’re also in charge of defining and implementing data standards and rules for the development and usage of data models. There is often some confusion surrounding the primary differences between data architects and engineers, but the visionary component is undoubtedly one of the most important.
Typical skills required to be a data architect include the ability to design data processing models to apply business models, create visualizations of important data entries and any connections, possess good communication skills in order to explain difficult-to-understand concepts to coworkers across teams, and the ability to ascertain and lay out the correct list of components required for a design system.
Data architects can expect to earn around six figures a year thanks to their ability to help businesses wade through the chaos of large amounts of unsynthesized data.
Data Engineer
In enormous data sets, data engineers hunt for patterns. They also create algorithms to assist corporations in extracting relevant information from large amounts of data.
A data engineer must be able to process data sets that are being developed, prepare data for modeling (prescriptive and predictive), search for hidden patterns in data, and provide data results feedback to all of a business’ stakeholders in a language they understand.
Data engineers, like their other data scientist counterparts, are an essential part of modern business and, therefore, command high starting salaries, often over $100,000 per year.
Infrastructure Architect
Infrastructure architects are in charge of creating and executing IT solutions that can support an organization’s technological infrastructure. They also resolve any security or performance issues that may affect the company.
Among their responsibilities are providing end-to-end system, network, and application troubleshooting; assisting with data backup and recovery; and creating and managing documentation for network analysis that is being updated.
Infrastructure architects earn over $100,000 per year and continue to be in high demand in companies with a more complex IT infrastructure. This, increasingly, is most medium to large companies, as they automate, deal with remote workforces, and try to manage the growing cybersecurity risks that abound. Experienced infrastructure architects, especially those with a cybersecurity specialization, will be in high demand from here on out.
Enterprise Architect
Enterprise architects assist businesses with the development of their IT infrastructure. They’re also responsible for the maintenance and upkeep of IT systems, networks, and services. As an enterprise architect, you must stay on top of emerging trends that might improve the efficiency of your company’s procedures.
Enterprise architects use a wide range of skills, including data leadership, in order to communicate with people of various technical abilities and problem-solving in order to collaborate with various different teams to implement solutions. Enterprise architects can easily earn over $120,000 per year, which can be augmented by different specializations and certifications, especially anything cybersecurity- and fraud-related.
Businesses are currently scrambling to find the talent required to build out the IT infrastructure that can meet future demands.
Applications Architect
Applications architects are in charge of the overall system’s monitoring. They must have a solid understanding of how each component interacts with one another and impacts business operations.
An applications architect is proficient in modeling systems, recognizing the importance of application integration, security, and object-oriented analysis and design development of an application. You can expect to earn well over $100,000 as an applications architect, working in industries like corporate IT or at software development firms.
Statistician
To evaluate and interpret data, statisticians employ a variety of statistical models and approaches, taking a company’s suggestions into the decision-making process. Statisticians are in charge of coming up with data collection design techniques, using computers to process data for statistical modeling and visual analysis, and developing experimental designs and sampling approaches.
Statisticians are not as highly paid as other data scientist specialists, but they will almost always be in high demand. Qualified statisticians are constantly in demand in industries such as health care, government, and physical science.
Employment prospects for statisticians and mathematicians are predicted to expand greatly in the coming years, especially as the models and algorithms used in business become more mathematically complex.
Data Analyst
Data analysts convert data into a format that firms can understand. A large part of the purpose of a data analyst is to provide businesses with a picture of their present performance. Business executives then utilize this feedback to make tactical decisions regarding the future of the company.
The typical responsibilities of a data analyst include things like working with management to determine how to prioritize informational requirements, creating reports using statistical approaches and techniques, locating information in a variety of sources, and data filtering and refinement for statistical and reporting purposes.
Data analysts are not as well-paid as their other data scientist counterparts, but they are still highly sought after as more and more companies look to improve how they use analytics. The ability to analyze data and help companies make better use of the often-disorganized and chaotic data they do have is worth paying for and is an easy skill to sell as a data scientist.
Conclusion
The current economy, to say nothing of the economy of the future, is entirely reliant on data analysis and competent data scientists. The economic reasons alone for pursuing a career in Data Science are compelling enough. It is also a highly dynamic career choice, with applications across industries that allow you to spend a lot of time thinking, solving problems, and providing actionable insight that has a real impact on the bottom line.
Best of all, Data Science is a discipline that will constantly provide you with opportunities to learn and upskill. New advances are constantly being made, which require advancing your understanding of your craft and industries. Data Science is the way of the future, and there is unlikely to be a dull moment as we make bigger and better use of data.